1 回答
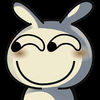
TA贡献1817条经验 获得超14个赞
这里有一些你可以尝试的事情。您可以通过更改 train_gen 来消除 Lambda 层,如下所示
rescaled3D_gen = tf.keras.preprocessing.image.ImageDataGenerator(rescale=1./255, zoom_range=0.2,shear_range=0.2, horizontal_flip=True,
preprocessing_function=tf.keras.applications.mobilenet_v2.preprocess_input)
您不需要 Lamda 调整大小图层,因为您在目录流中指定了目标大小。在 val_gen 中,您有 shuffle=True。这将打乱每个时期的验证图像顺序。为了保持一致性,最好将其设置为 False。在 mobilenet 的代码中,您有 include_top=True 和 pooling='avg' 当 include_top 为 True 时,池参数将被忽略。设置 include_top=True 会使模型的顶层具有 1000 个节点的密集层和 softmax 激活函数。我会设置 include_top=False。这样,mobilenet 的输出就是一个全局池化层,可以直接为您的密集分类层提供数据。在生成器中设置 class_mode='binary'。但在 model.compile 中,您将损失设置为稀疏_分类_交叉熵。这可以工作,但使用 loss=BinaryCrossentropy 进行编译会更好。为了保持一致性,最好每个时期只检查一次验证样本。为此,应选择批量大小,使验证样本/batch_size 为整数,并使用该整数作为验证步骤数。下面的代码将为您做到这一点。
b_max=80 # set this to the maximum batch size you will allow based on memory capacity
length=val_gen.samples
batch_size=sorted([int(length/n) for n in range(1,length+1) if length % n ==0 and length/n<=b_max],reverse=True)[0]
val_steps=int(length/batch_size)
更改验证批量大小可能会改变验证损失和准确性的结果。一般来说,较大的批量大小会导致损失波动较小,但可能会导致陷入局部最小值的可能性较高。尝试这些更改,看看结果的差异是否较小。
添加回答
举报