1 回答
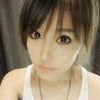
TA贡献1775条经验 获得超11个赞
我的建议是定义编码器和解码器的结构(get_encoder,get_decoder)。在整个模型的训练之后,我们的想法是创建一个新的解码器架构(通过get_decoder),我们可以用解码器训练的权重来填充它
pythonic 来说你可以用这种方式做到这一点......
def get_crop_shape(target, refer):
# width, the 3rd dimension
cw = (target.get_shape()[2] - refer.get_shape()[2])
assert (cw >= 0)
if cw % 2 != 0:
cw1, cw2 = cw // 2, cw // 2 + 1
else:
cw1, cw2 = cw // 2, cw // 2
# height, the 2nd dimension
ch = (target.get_shape()[1] - refer.get_shape()[1])
assert (ch >= 0)
if ch % 2 != 0:
ch1, ch2 = ch // 2, ch // 2 + 1
else:
ch1, ch2 = ch // 2, ch // 2
return (ch1, ch2), (cw1, cw2)
def get_encoder(img_shape):
inp = Input(shape=img_shape)
conv1 = Conv2D(64, (5, 5), activation='relu', padding='same', data_format="channels_last", name='conv1_1')(inp)
conv1 = Conv2D(64, (5, 5), activation='relu', padding='same', data_format="channels_last", name='conv1_2')(conv1)
pool1 = MaxPooling2D(pool_size=(2, 2), data_format="channels_last", name='pool1')(conv1)
conv2 = Conv2D(96, (3, 3), activation='relu', padding='same', data_format="channels_last", name='conv2_1')(pool1)
conv2 = Conv2D(96, (3, 3), activation='relu', padding='same', data_format="channels_last", name='conv2_2')(conv2)
pool2 = MaxPooling2D(pool_size=(2, 2), data_format="channels_last", name='pool2')(conv2)
conv3 = Conv2D(128, (3, 3), activation='relu', padding='same', data_format="channels_last", name='conv3_1')(pool2)
conv3 = Conv2D(128, (3, 3), activation='relu', padding='same', data_format="channels_last", name='conv3_2')(conv3)
pool3 = MaxPooling2D(pool_size=(2, 2), data_format="channels_last", name='pool3')(conv3)
conv4 = Conv2D(256, (3, 3), activation='relu', padding='same', data_format="channels_last", name='conv4_1')(pool3)
conv4 = Conv2D(256, (4, 4), activation='relu', padding='same', data_format="channels_last", name='conv4_2')(conv4)
pool4 = MaxPooling2D(pool_size=(2, 2), data_format="channels_last", name='pool4')(conv4)
conv5 = Conv2D(512, (3, 3), activation='relu', padding='same', data_format="channels_last", name='conv5_1')(pool4)
conv5 = Conv2D(512, (3, 3), activation='relu', padding='same', data_format="channels_last", name='conv5_2')(conv5)
return conv5,conv4,conv3,conv2,conv1,inp
def get_decoder(convs):
conv5,conv4,conv3,conv2,conv1,inputs = convs
up_conv5 = UpSampling2D(size=(2, 2), data_format="channels_last", name='up_conv5')(conv5)
ch, cw = get_crop_shape(conv4, up_conv5)
crop_conv4 = Cropping2D(cropping=(ch, cw), data_format="channels_last", name='crop_conv4')(conv4)
up6 = concatenate([up_conv5, crop_conv4])
conv6 = Conv2D(256, (3, 3), activation='relu', padding='same', data_format="channels_last", name='conv6_1')(up6)
conv6 = Conv2D(256, (3, 3), activation='relu', padding='same', data_format="channels_last", name='conv6_2')(conv6)
up_conv6 = UpSampling2D(size=(2, 2), data_format="channels_last", name='up_conv6')(conv6)
ch, cw = get_crop_shape(conv3, up_conv6)
crop_conv3 = Cropping2D(cropping=(ch, cw), data_format="channels_last", name='crop_conv3')(conv3)
up7 = concatenate([up_conv6, crop_conv3])
conv7 = Conv2D(128, (3, 3), activation='relu', padding='same', data_format="channels_last", name='conv7_1')(up7)
conv7 = Conv2D(128, (3, 3), activation='relu', padding='same', data_format="channels_last", name='conv7_2')(conv7)
up_conv7 = UpSampling2D(size=(2, 2), data_format="channels_last", name='up_conv7')(conv7)
ch, cw = get_crop_shape(conv2, up_conv7)
crop_conv2 = Cropping2D(cropping=(ch, cw), data_format="channels_last", name='crop_conv2')(conv2)
up8 = concatenate([up_conv7, crop_conv2])
conv8 = Conv2D(96, (3, 3), activation='relu', padding='same', data_format="channels_last", name='conv8_1')(up8)
conv8 = Conv2D(96, (3, 3), activation='relu', padding='same', data_format="channels_last", name='conv8_2')(conv8)
up_conv8 = UpSampling2D(size=(2, 2), data_format="channels_last", name='up_conv8')(conv8)
ch, cw = get_crop_shape(conv1, up_conv8)
crop_conv1 = Cropping2D(cropping=(ch, cw), data_format="channels_last", name='crop_conv1')(conv1)
up9 = concatenate([up_conv8, crop_conv1])
conv9 = Conv2D(64, (3, 3), activation='relu', padding='same', data_format="channels_last", name='conv9_1')(up9)
conv9 = Conv2D(64, (3, 3), activation='relu', padding='same', data_format="channels_last", name='conv9_2')(conv9)
ch, cw = get_crop_shape(inputs, conv9)
conv9 = ZeroPadding2D(padding=(ch, cw), data_format="channels_last", name='conv9_3')(conv9)
conv10 = Conv2D(1, (1, 1), activation='sigmoid', data_format="channels_last", name='conv10_1')(conv9)
return conv10
def get_unet(img_shape = (200,200,1)):
enc = get_encoder(img_shape)
dec = get_decoder(enc)
model = Model(inputs=enc[-1], outputs=dec)
return model
创建整个模型并拟合
img_shape = (200,200,1)
old_model = get_unet(img_shape)
# old_model.compile(...)
# old_model.fit(...)
一如既往地提取编码器
# extract encoder
first_encoder_layer = 0
last_encoder_layer = 14
encoder_output_layer = [14, 11, 8, 5, 2, 0]
encoder = Model(inputs=old_model.layers[first_encoder_layer].input,
outputs=[old_model.layers[l].output for l in encoder_output_layer],
name='encoder')
encoder.summary()
创建解码器结构并分配训练后的权重
# extract decoder fitted weights
restored_w = []
for w in old_model.layers[last_encoder_layer + 1:]:
restored_w.extend(w.get_weights())
# reconstruct decoder architecture setting the fitted weights
new_inp = [Input(l.shape[1:]) for l in get_encoder(img_shape)]
new_dec = get_decoder(new_inp)
decoder = Model(new_inp, new_dec)
decoder.set_weights(restored_w)
decoder.summary()
返回预测
# generate random images
n_images = 20
X = np.random.uniform(0,1, (n_images,200,200,1)).astype('float32')
# get encoder predictions
pred_encoder = encoder.predict(X)
print([p.shape for p in pred_encoder])
# get decoder predictions
pred_decoder = decoder.predict(pred_encoder)
print(pred_decoder.shape)
添加回答
举报