2 回答
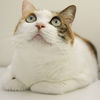
TA贡献1921条经验 获得超9个赞
这一点也不奇怪~网络问题
import networkx as nx
#we need to handle the miss value first , we fill it with same row, so that we did not calssed them into wrong group
df['key1']=df['group1_id'].fillna(df['group2_id'])
df['key2']=df['group2_id'].fillna(df['group1_id'])
# here we start to create the network
G=nx.from_pandas_edgelist(df, 'key1', 'key2')
l=list(nx.connected_components(G))
L=[dict.fromkeys(y,x) for x, y in enumerate(l)]
d={k: v for d in L for k, v in d.items()}
# we using above dict to map the same group into the same one in order to groupby them
out=df.groupby(df.key1.map(d)).agg(objs = ('obj',list) , Count = ('obj','count'), g1= ('group1_id', lambda x : set(x[x.notnull()].tolist())), g2= ('group2_id', lambda x : set(x[x.notnull()].tolist())))
# notice here I did not conver the composite id into string format , I keep them into different columns which more easy to understand
Out[53]:
objs Count g1 g2
key1
0 [Ball, Balloon, Bottle, Thought] 4 {92, 3} {7}
1 [Person] 1 {14} {11}
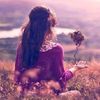
TA贡献1815条经验 获得超6个赞
这里有一个更详细的解决方案,我为分组集合构建了“第一个键”的映射:
# using four id fields instead of 2
grouping_fields = ['group1_id', 'group2_id', 'group3_id', 'group4_id']
id_fields = df.loc[df[grouping_fields].notnull().any(axis=1), grouping_fields]
# build a set of all similarly-grouped items
# and use the 'first seen' as the grouping key for that
FIRST_SEEN_TO_ALL = defaultdict(set)
KEY_TO_FIRST_SEEN = {}
for row in id_fields.to_dict('records'):
# why doesn't nan fall out in a boolean check?
keys = [id for id in row.values() if id and (str(id) != 'nan')]
row_id = keys[0]
for key in keys:
if (row_id != key) or (key not in KEY_TO_FIRST_SEEN):
KEY_TO_FIRST_SEEN[key] = row_id
first_seen_key = row_id
else:
first_seen_key = KEY_TO_FIRST_SEEN[key]
FIRST_SEEN_TO_ALL[first_seen_key].add(key)
def fetch_group_id(row):
keys = filter(None, row.to_dict().values())
for key in keys:
first_seen_key = KEY_TO_FIRST_SEEN.get(key)
if first_seen_key:
return first_seen_key
df['group_super'] = df[grouping_fields].apply(fetch_group_id, axis=1)
添加回答
举报