2 回答
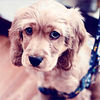
TA贡献1883条经验 获得超3个赞
您可能正在寻找实体提及而不是 NER 标签。例如使用简单 API:
new Sentence("Jimi Hendrix was the greatest").nerTags()
[PERSON, PERSON, O, O, O]
new Sentence("Jimi Hendrix was the greatest").mentions()
[Jimi Hendrix]
StanfordCoreNLP上面的链接有一个使用旧管道的传统非简单 API 的示例
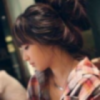
TA贡献1818条经验 获得超7个赞
这是完整的 Java API 示例,其中有一个关于实体提及的部分:
import edu.stanford.nlp.coref.data.CorefChain;
import edu.stanford.nlp.ling.*;
import edu.stanford.nlp.ie.util.*;
import edu.stanford.nlp.pipeline.*;
import edu.stanford.nlp.semgraph.*;
import edu.stanford.nlp.trees.*;
import java.util.*;
public class BasicPipelineExample {
public static String text = "Joe Smith was born in California. " +
"In 2017, he went to Paris, France in the summer. " +
"His flight left at 3:00pm on July 10th, 2017. " +
"After eating some escargot for the first time, Joe said, \"That was delicious!\" " +
"He sent a postcard to his sister Jane Smith. " +
"After hearing about Joe's trip, Jane decided she might go to France one day.";
public static void main(String[] args) {
// set up pipeline properties
Properties props = new Properties();
// set the list of annotators to run
props.setProperty("annotators", "tokenize,ssplit,pos,lemma,ner,parse,depparse,coref,kbp,quote");
// set a property for an annotator, in this case the coref annotator is being set to use the neural algorithm
props.setProperty("coref.algorithm", "neural");
// build pipeline
StanfordCoreNLP pipeline = new StanfordCoreNLP(props);
// create a document object
CoreDocument document = new CoreDocument(text);
// annnotate the document
pipeline.annotate(document);
// examples
// 10th token of the document
CoreLabel token = document.tokens().get(10);
System.out.println("Example: token");
System.out.println(token);
System.out.println();
// text of the first sentence
String sentenceText = document.sentences().get(0).text();
System.out.println("Example: sentence");
System.out.println(sentenceText);
System.out.println();
// second sentence
CoreSentence sentence = document.sentences().get(1);
// list of the part-of-speech tags for the second sentence
List<String> posTags = sentence.posTags();
System.out.println("Example: pos tags");
System.out.println(posTags);
System.out.println();
// list of the ner tags for the second sentence
List<String> nerTags = sentence.nerTags();
System.out.println("Example: ner tags");
System.out.println(nerTags);
System.out.println();
// constituency parse for the second sentence
Tree constituencyParse = sentence.constituencyParse();
System.out.println("Example: constituency parse");
System.out.println(constituencyParse);
System.out.println();
// dependency parse for the second sentence
SemanticGraph dependencyParse = sentence.dependencyParse();
System.out.println("Example: dependency parse");
System.out.println(dependencyParse);
System.out.println();
// kbp relations found in fifth sentence
List<RelationTriple> relations =
document.sentences().get(4).relations();
System.out.println("Example: relation");
System.out.println(relations.get(0));
System.out.println();
// entity mentions in the second sentence
List<CoreEntityMention> entityMentions = sentence.entityMentions();
System.out.println("Example: entity mentions");
System.out.println(entityMentions);
System.out.println();
// coreference between entity mentions
CoreEntityMention originalEntityMention = document.sentences().get(3).entityMentions().get(1);
System.out.println("Example: original entity mention");
System.out.println(originalEntityMention);
System.out.println("Example: canonical entity mention");
System.out.println(originalEntityMention.canonicalEntityMention().get());
System.out.println();
// get document wide coref info
Map<Integer, CorefChain> corefChains = document.corefChains();
System.out.println("Example: coref chains for document");
System.out.println(corefChains);
System.out.println();
// get quotes in document
List<CoreQuote> quotes = document.quotes();
CoreQuote quote = quotes.get(0);
System.out.println("Example: quote");
System.out.println(quote);
System.out.println();
// original speaker of quote
// note that quote.speaker() returns an Optional
System.out.println("Example: original speaker of quote");
System.out.println(quote.speaker().get());
System.out.println();
// canonical speaker of quote
System.out.println("Example: canonical speaker of quote");
System.out.println(quote.canonicalSpeaker().get());
System.out.println();
}
}
添加回答
举报