1 回答
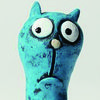
TA贡献1799条经验 获得超9个赞
您错过了在模型中添加激活函数。对于任何逼近非线性函数的神经网络,非线性激活函数是必需的。
一个神经元(没有激活的线性回归单元)可以表示为
其中,f(x; W,b)表示由W 和 b参数化的x的函数。
考虑单个输入、2 个隐藏神经元、单个输出网络:
隐藏的神经元是:n1 = w1.x + b1** and **n2 = w2.x + b2
输出神经元是:n3 = w3.n1 + w4.n2 + b3
代入n1
并n2
输入输出神经元:
n3 = w3(w1.x + b1) + w4(w2.x + b2) + b3** n3 = w1w3x + b1 + w2w4x + b2 + b3** n3 = x(w1w3 + w2w4) + (b1 + b2 + b3) n3 = Wx + B
其中W 和 B是常数W = w1w3+w2w4
和B = b1+b2+b3
。
所以最后这neural network
归结为一个Linear regression unit。因此,这不能逼近任何非线性函数,因为线性方程的线性组合始终是线性方程。
所以只需要引入隐藏神经元的激活函数。它会起作用的。
这是修改后的代码:
# f(x) = 0.22*x*x*x-0.6*x*x+0.4*x-0.5
import tensorflow as tf
import numpy as np
import logging
logger = tf.get_logger()
logger.setLevel(logging.ERROR)
x = np.array([-5,-4.8,-4.6,-4.4,-4.2,-4,-3.8,-3.6,-3.4,-3.2,-3,-2.8,-2.6,-2.4,-2.2,-2,-1.8,-1.6,-1.4,-1.2,-1,-0.8,-0.6,-0.4,-0.2,0,0.2,0.4,0.6,0.8,1,1.2,1.4,1.6,1.8,2,2.2,2.4,2.6,2.8,3,3.2,3.4,3.6,3.8,4,4.2,4.4,4.6,4.8,5], dtype=float)
y = np.array([-45,-40.57424,-36.44992,-32.61648,-29.06336,-25.78,-22.75584,-19.98032,-17.44288,-15.13296,-13.04,-11.15344,-9.46272,-7.95728,-6.62656,-5.46,-4.44704,-3.57712,-2.83968,-2.22416,-1.72,-1.31664,-1.00352,-0.77008,-0.60576,-0.5,-0.44224,-0.42192,-0.42848,-0.45136,-0.48,-0.50384,-0.51232,-0.49488,-0.44096,-0.34,-0.18144,0.04528,0.350720000000001,0.745439999999999,1.24,1.84496,2.57088,3.42832,4.42784,5.58,6.89536,8.38448,10.05792,11.92624,14], dtype=float)
for i,c in enumerate(x):
print("x = {}, y = {}".format(x[i], y[i]))
#######################added activation#####################################
l0 = tf.keras.layers.Dense(units=4, activation='relu', input_shape=[1])
l1 = tf.keras.layers.Dense(units=10, activation='relu',)
############################################################################
l2 = tf.keras.layers.Dense(units=1)
model = tf.keras.Sequential([l0, l1, l2])
model.compile(optimizer=tf.keras.optimizers.Adam(learning_rate=1e-1), loss='mean_squared_error', metrics=['mean_squared_error'])
history = model.fit(x, y, epochs=10000, verbose=1)
print("Finished training the model")
import matplotlib.pyplot as plt
plt.xlabel('Epoch Number')
plt.ylabel("Loss Magnitude")
plt.plot(history.history['loss'])
plt.show()
print("For x = -5 (nom = -45), y = {} ".format(model.predict([-5]))) # should be -45
print("For x = -3 (nom = -13.4), y = {} ".format(model.predict([-3]))) # should be -13.4
print("For x = -2.4 (nom = -7.96), y = {} ".format(model.predict([-2.4]))) # should be -7.96
print("For x = -1.4 (nom = -2.84), y = {} ".format(model.predict([-1.4]))) # should be -2.84
print("For x = 0 (nom = -0.5), y = {} ".format(model.predict([0]))) # should be -0.5
print("For x = 1.2 (nom = -0.50), y = {} ".format(model.predict([1.2]))) # should be -0.50
print("For x = 3.4 (nom = 2.57), y = {} ".format(model.predict([3.4]))) # should be 2.57
print("For x = 4.8 (nom = 11.93), y = {} ".format(model.predict([4.8]))) # should be 11.93
print("Model predicts that for x = 3.18, y = {} ".format(model.predict([3.18])))
以下是结果:
.
.
.
.
Epoch 9997/10000
2/2 [==============================] - 0s 2ms/step - loss: 0.2931 - mean_squared_error: 0.2931
Epoch 9998/10000
2/2 [==============================] - 0s 997us/step - loss: 0.2993 - mean_squared_error: 0.2993
Epoch 9999/10000
2/2 [==============================] - 0s 498us/step - loss: 0.2872 - mean_squared_error: 0.2872
Epoch 10000/10000
2/2 [==============================] - 0s 498us/step - loss: 0.2598 - mean_squared_error: 0.2598
Finished training the model
For x = -5 (nom = -45), y = [[-44.53207]]
For x = -3 (nom = -13.4), y = [[-12.643011]]
For x = -2.4 (nom = -7.96), y = [[-8.224297]]
For x = -1.4 (nom = -2.84), y = [[-0.7255349]]
For x = 0 (nom = -0.5), y = [[-0.7255349]]
For x = 1.2 (nom = -0.50), y = [[-0.7255349]]
For x = 3.4 (nom = 2.57), y = [[2.8460937]]
For x = 4.8 (nom = 11.93), y = [[12.013783]]
Model predicts that for x = 3.18, y = [[1.9653977]]
损耗曲线:
添加回答
举报