1 回答
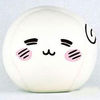
TA贡献1874条经验 获得超12个赞
给定图:kmeans 聚类质心,其中
centers
是一维。该centers
数组有一个(3, 2)
形状,带有x
as(3, 1)
和y
as(3, 1)
。针对这个一维中心展示的方法,已经适用于为中心的七个维度生成一个解决方案,由模型为这个问题生成。
此问题中模型的返回值有七个维度,其中
centers
的形状是 7 组和值。(7, 14)
14
x
y
这个解决方案回答了这个问题,How to plot the clusters & centers?
它不提供对模型结果的评论或解释,这需要在SE: Cross Validated或SE: Data Science中提出不同的问题。
# uses the imports as shown in the question
from matplotlib.patches import Rectangle, Patch # for creating a legend
from matplotlib.lines import Line2D
# beginning with
features = trans.fit_transform(features)
# create the model and fit it to features
kmeans_model2 = KMeans(n_clusters=7, init='k-means++', random_state=0).fit(features)
# find the centers; there are 7
centers = np.array(kmeans_model2.cluster_centers_)
# unique markers for the labels
markers = ['o', 'v', 's', '*', 'p', 'd', 'h']
# get the model labels
labels = kmeans_model2.labels_
labels_unique = set(labels)
# unique colors for each label
colors = sns.color_palette('husl', n_colors=len(labels_unique))
# color map with labels and colors
cmap = dict(zip(labels_unique, colors))
# plot
# iterate through each group of 2 centers
for j in range(0, len(centers)*2, 2):
plt.figure(figsize=(6, 6))
x_features = features[:, j]
y_features = features[:, j+1]
x_centers = centers[:, j]
y_centers = centers[:, j+1]
# add the data for each label to the plot
for i, l in enumerate(labels):
# print(f'Label: {l}') # uncomment as needed
# print(f'feature x coordinates for label:\n{x_features[i]}') # uncomment as needed
# print(f'feature y coordinates for label:\n{y_features[i]}') # uncomment as needed
plt.plot(x_features[i], y_features[i], color=colors[l], marker=markers[l], alpha=0.5)
# print values for given plot, rounded for easier interpretation; all 4 can be commented out
print(f'feature labels:\n{list(labels)}')
print(f'x_features:\n{list(map(lambda x: round(x, 3), x_features))}')
print(f'y_features:\n{list(map(lambda x: round(x, 3), y_features))}')
print(f'x_centers:\n{list(map(lambda x: round(x, 3), x_centers))}')
print(f'y_centers:\n{list(map(lambda x: round(x, 3), y_centers))}')
# add the centers
# this loop is to color the center marker to correspond to the color of the corresponding label.
for k in range(len(centers)):
plt.scatter(x_centers[k], y_centers[k], marker="X", color=colors[k])
# title
plt.title(f'Features: Dimension {int(j/2)}')
# create the rectangles for the legend
patches = [Patch(color=v, label=k) for k, v in cmap.items()]
# create centers marker for the legend
black_x = Line2D([], [], color='k', marker='X', linestyle='None', label='centers', markersize=10)
# add the legend
plt.legend(title='Labels', handles=patches + [black_x], bbox_to_anchor=(1.04, 0.5), loc='center left', borderaxespad=0, fontsize=15)
plt.show()
绘图输出
许多绘制的特征具有重叠的值和中心。
和的
x
和y
值已被打印出来,以便更容易地看到重叠,并确认绘制的值。features
centers
print
当不再需要时,可以注释掉或删除负责的行。
特征 0
feature labels:
[6, 1, 1, 1, 5, 5, 3, 4, 1, 0, 1, 5, 5, 1, 1, 1, 1, 1, 4, 1, 2, 0, 1, 3, 3, 4, 2, 2, 4, 3, 3, 2, 6, 3, 1, 2, 4, 6, 1, 4, 4, 1, 4, 5, 3, 1, 1, 1, 1, 1, 0, 1, 5, 5, 1, 1, 3, 3, 3, 1, 3, 1, 3, 3, 0, 1, 2, 2, 2, 6]
x_features:
[0.0, 1.0, 1.0, 1.0, 1.0, 1.0, 1.0, 1.0, 1.0, 1.0, 1.0, 1.0, 1.0, 1.0, 1.0, 1.0, 1.0, 1.0, 1.0, 1.0, 0.0, 1.0, 1.0, 1.0, 1.0, 1.0, 0.0, 0.0, 1.0, 1.0, 1.0, 0.0, 0.0, 1.0, 1.0, 0.0, 1.0, 0.0, 1.0, 1.0, 1.0, 1.0, 1.0, 1.0, 1.0, 1.0, 1.0, 1.0, 1.0, 1.0, 1.0, 1.0, 1.0, 1.0, 1.0, 1.0, 1.0, 1.0, 1.0, 1.0, 1.0, 1.0, 1.0, 1.0, 1.0, 1.0, 0.0, 0.0, 0.0, 0.0]
y_features:
[1.0, 0.0, 0.0, 0.0, 0.0, 0.0, 0.0, 0.0, 0.0, 0.0, 0.0, 0.0, 0.0, 0.0, 0.0, 0.0, 0.0, 0.0, 0.0, 0.0, 1.0, 0.0, 0.0, 0.0, 0.0, 0.0, 1.0, 1.0, 0.0, 0.0, 0.0, 1.0, 1.0, 0.0, 0.0, 1.0, 0.0, 1.0, 0.0, 0.0, 0.0, 0.0, 0.0, 0.0, 0.0, 0.0, 0.0, 0.0, 0.0, 0.0, 0.0, 0.0, 0.0, 0.0, 0.0, 0.0, 0.0, 0.0, 0.0, 0.0, 0.0, 0.0, 0.0, 0.0, 0.0, 0.0, 1.0, 1.0, 1.0, 1.0]
x_centers:
[1.0, 1.0, 0.0, 1.0, 1.0, 1.0, 0.0]
y_centers:
[0.0, 0.0, 1.0, 0.0, -0.0, -0.0, 1.0]
特点 1
feature labels:
[6, 1, 1, 1, 5, 5, 3, 4, 1, 0, 1, 5, 5, 1, 1, 1, 1, 1, 4, 1, 2, 0, 1, 3, 3, 4, 2, 2, 4, 3, 3, 2, 6, 3, 1, 2, 4, 6, 1, 4, 4, 1, 4, 5, 3, 1, 1, 1, 1, 1, 0, 1, 5, 5, 1, 1, 3, 3, 3, 1, 3, 1, 3, 3, 0, 1, 2, 2, 2, 6]
x_features:
[0.0, 0.0, 0.0, 0.0, 0.0, 0.0, 0.0, 0.0, 0.0, 1.0, 0.0, 0.0, 0.0, 0.0, 0.0, 0.0, 0.0, 0.0, 0.0, 0.0, 0.0, 1.0, 0.0, 0.0, 0.0, 0.0, 0.0, 0.0, 0.0, 0.0, 0.0, 0.0, 0.0, 0.0, 0.0, 0.0, 0.0, 0.0, 0.0, 0.0, 0.0, 0.0, 0.0, 0.0, 0.0, 0.0, 0.0, 0.0, 0.0, 0.0, 1.0, 0.0, 0.0, 0.0, 0.0, 0.0, 0.0, 0.0, 0.0, 0.0, 0.0, 0.0, 0.0, 0.0, 1.0, 0.0, 0.0, 0.0, 0.0, 0.0]
y_features:
[1.0, 1.0, 1.0, 1.0, 0.0, 0.0, 0.0, 0.0, 1.0, 0.0, 1.0, 0.0, 0.0, 1.0, 1.0, 1.0, 1.0, 1.0, 0.0, 1.0, 0.0, 0.0, 1.0, 0.0, 0.0, 0.0, 0.0, 0.0, 0.0, 0.0, 0.0, 0.0, 1.0, 0.0, 1.0, 0.0, 0.0, 1.0, 1.0, 0.0, 0.0, 1.0, 0.0, 0.0, 0.0, 1.0, 1.0, 1.0, 1.0, 1.0, 0.0, 1.0, 0.0, 0.0, 1.0, 1.0, 0.0, 0.0, 0.0, 1.0, 0.0, 1.0, 0.0, 0.0, 0.0, 1.0, 0.0, 0.0, 0.0, 1.0]
x_centers:
[1.0, -0.0, -0.0, -0.0, -0.0, 0.0, 0.0]
y_centers:
[0.0, 1.0, 0.0, -0.0, 0.0, 0.0, 1.0]
特征2
feature labels:
[6, 1, 1, 1, 5, 5, 3, 4, 1, 0, 1, 5, 5, 1, 1, 1, 1, 1, 4, 1, 2, 0, 1, 3, 3, 4, 2, 2, 4, 3, 3, 2, 6, 3, 1, 2, 4, 6, 1, 4, 4, 1, 4, 5, 3, 1, 1, 1, 1, 1, 0, 1, 5, 5, 1, 1, 3, 3, 3, 1, 3, 1, 3, 3, 0, 1, 2, 2, 2, 6]
x_features:
[0.0, 0.0, 0.0, 0.0, 0.0, 0.0, 1.0, 0.0, 0.0, 0.0, 0.0, 0.0, 0.0, 0.0, 0.0, 0.0, 0.0, 0.0, 0.0, 0.0, 0.0, 0.0, 0.0, 1.0, 1.0, 0.0, 0.0, 0.0, 0.0, 1.0, 1.0, 0.0, 0.0, 1.0, 0.0, 0.0, 0.0, 0.0, 0.0, 0.0, 0.0, 0.0, 0.0, 0.0, 1.0, 0.0, 0.0, 0.0, 0.0, 0.0, 0.0, 0.0, 0.0, 0.0, 0.0, 0.0, 1.0, 1.0, 1.0, 0.0, 1.0, 0.0, 1.0, 1.0, 0.0, 0.0, 0.0, 0.0, 1.0, 0.0]
y_features:
[0.0, 0.0, 0.0, 0.0, 1.0, 1.0, 0.0, 0.0, 0.0, 0.0, 0.0, 1.0, 1.0, 0.0, 0.0, 0.0, 0.0, 0.0, 0.0, 0.0, 0.0, 0.0, 0.0, 0.0, 0.0, 0.0, 0.0, 0.0, 0.0, 0.0, 0.0, 0.0, 0.0, 0.0, 0.0, 0.0, 0.0, 0.0, 0.0, 0.0, 0.0, 0.0, 0.0, 1.0, 0.0, 0.0, 0.0, 0.0, 0.0, 0.0, 0.0, 0.0, 1.0, 1.0, 0.0, 0.0, 0.0, 0.0, 0.0, 0.0, 0.0, 0.0, 0.0, 0.0, 0.0, 0.0, 0.0, 0.0, 0.0, 0.0]
x_centers:
[0.0, -0.0, 0.125, 1.0, 0.0, 0.0, 0.0]
y_centers:
[0.0, -0.0, 0.0, 0.0, 0.0, 1.0, 0.0]
特色三
feature labels:
[6, 1, 1, 1, 5, 5, 3, 4, 1, 0, 1, 5, 5, 1, 1, 1, 1, 1, 4, 1, 2, 0, 1, 3, 3, 4, 2, 2, 4, 3, 3, 2, 6, 3, 1, 2, 4, 6, 1, 4, 4, 1, 4, 5, 3, 1, 1, 1, 1, 1, 0, 1, 5, 5, 1, 1, 3, 3, 3, 1, 3, 1, 3, 3, 0, 1, 2, 2, 2, 6]
x_features:
[0.0, 0.0, 0.0, 0.0, 0.0, 0.0, 0.0, 1.0, 0.0, 0.0, 0.0, 0.0, 0.0, 0.0, 0.0, 0.0, 0.0, 0.0, 1.0, 0.0, 1.0, 0.0, 0.0, 0.0, 0.0, 1.0, 1.0, 1.0, 1.0, 0.0, 0.0, 1.0, 0.0, 0.0, 0.0, 1.0, 1.0, 0.0, 0.0, 1.0, 1.0, 0.0, 1.0, 0.0, 0.0, 0.0, 0.0, 0.0, 0.0, 0.0, 0.0, 0.0, 0.0, 0.0, 0.0, 0.0, 0.0, 0.0, 0.0, 0.0, 0.0, 0.0, 0.0, 0.0, 0.0, 0.0, 1.0, 1.0, 0.0, 0.0]
y_features:
[0.298, 0.193, 0.18, 0.336, 0.181, 0.174, 0.197, 0.23, 0.175, 0.212, 0.196, 0.186, 0.2, 0.15, 0.141, 0.304, 0.108, 0.101, 0.304, 0.105, 0.459, 0.18, 0.16, 0.224, 0.216, 0.246, 0.139, 0.111, 0.227, 0.177, 0.159, 0.25, 0.298, 0.223, 0.335, 0.431, 0.17, 0.381, 0.255, 0.222, 0.296, 0.156, 0.202, 0.145, 0.195, 0.15, 0.141, 0.18, 0.336, 0.175, 0.212, 0.196, 0.186, 0.2, 0.15, 0.141, 0.177, 0.177, 0.177, 0.177, 0.177, 0.177, 0.224, 0.224, 0.18, 0.16, 0.222, 0.202, 0.18, 0.336]
x_centers:
[0.0, -0.0, 0.875, -0.0, 1.0, 0.0, 0.0]
y_centers:
[0.196, 0.188, 0.249, 0.196, 0.237, 0.182, 0.328]
特点 4
feature labels:
[6, 1, 1, 1, 5, 5, 3, 4, 1, 0, 1, 5, 5, 1, 1, 1, 1, 1, 4, 1, 2, 0, 1, 3, 3, 4, 2, 2, 4, 3, 3, 2, 6, 3, 1, 2, 4, 6, 1, 4, 4, 1, 4, 5, 3, 1, 1, 1, 1, 1, 0, 1, 5, 5, 1, 1, 3, 3, 3, 1, 3, 1, 3, 3, 0, 1, 2, 2, 2, 6]
x_features:
[0.712, 0.741, 0.763, 0.704, 0.749, 0.741, 0.754, 0.735, 0.744, 0.738, 0.743, 0.747, 0.758, 0.759, 0.749, 0.714, 0.766, 0.748, 0.728, 0.755, 0.681, 0.752, 0.762, 0.734, 0.721, 0.747, 0.749, 0.756, 0.737, 0.748, 0.742, 0.724, 0.712, 0.733, 0.73, 0.688, 0.722, 0.705, 0.777, 0.749, 0.733, 0.744, 0.733, 0.764, 0.739, 0.76, 0.749, 0.763, 0.704, 0.744, 0.738, 0.743, 0.747, 0.758, 0.759, 0.749, 0.748, 0.748, 0.748, 0.748, 0.748, 0.748, 0.734, 0.734, 0.752, 0.762, 0.749, 0.733, 0.763, 0.704]
y_features:
[0.614, 0.636, 0.612, 0.601, 0.631, 0.64, 0.62, 0.624, 0.636, 0.633, 0.632, 0.63, 0.61, 0.629, 0.641, 0.616, 0.629, 0.65, 0.601, 0.644, 0.539, 0.628, 0.623, 0.627, 0.65, 0.603, 0.641, 0.641, 0.616, 0.632, 0.648, 0.631, 0.614, 0.624, 0.58, 0.562, 0.666, 0.587, 0.565, 0.616, 0.591, 0.646, 0.642, 0.625, 0.631, 0.629, 0.641, 0.612, 0.601, 0.636, 0.633, 0.632, 0.63, 0.61, 0.629, 0.641, 0.632, 0.632, 0.632, 0.632, 0.632, 0.632, 0.627, 0.627, 0.628, 0.623, 0.616, 0.642, 0.612, 0.601]
x_centers:
[0.745, 0.747, 0.73, 0.741, 0.735, 0.752, 0.708]
y_centers:
[0.63, 0.625, 0.611, 0.632, 0.62, 0.625, 0.604]
特点 5
feature labels:
[6, 1, 1, 1, 5, 5, 3, 4, 1, 0, 1, 5, 5, 1, 1, 1, 1, 1, 4, 1, 2, 0, 1, 3, 3, 4, 2, 2, 4, 3, 3, 2, 6, 3, 1, 2, 4, 6, 1, 4, 4, 1, 4, 5, 3, 1, 1, 1, 1, 1, 0, 1, 5, 5, 1, 1, 3, 3, 3, 1, 3, 1, 3, 3, 0, 1, 2, 2, 2, 6]
x_features:
[0.164, 0.096, 0.103, 0.171, 0.091, 0.106, 0.094, 0.132, 0.105, 0.098, 0.102, 0.101, 0.115, 0.079, 0.095, 0.135, 0.075, 0.088, 0.126, 0.063, 0.186, 0.088, 0.075, 0.134, 0.107, 0.134, 0.09, 0.072, 0.16, 0.097, 0.073, 0.123, 0.165, 0.154, 0.133, 0.158, 0.084, 0.11, 0.105, 0.1, 0.164, 0.075, 0.1, 0.075, 0.135, 0.069, 0.095, 0.103, 0.171, 0.105, 0.098, 0.102, 0.101, 0.115, 0.079, 0.095, 0.097, 0.097, 0.097, 0.097, 0.097, 0.097, 0.134, 0.134, 0.088, 0.075, 0.1, 0.1, 0.103, 0.171]
y_features:
[0.001, 0.002, 0.001, 0.001, 0.001, 0.002, 0.002, 0.001, 0.001, 0.001, 0.001, 0.005, 0.002, 0.001, 0.002, 0.001, 0.002, 0.001, 0.001, 0.002, 0.0, 0.001, 0.001, 0.002, 0.0, 0.001, 0.001, 0.002, 0.002, 0.002, 0.0, 0.001, 0.001, 0.001, 0.004, 0.004, 0.001, 0.002, 0.001, 0.001, 0.002, 0.0, 0.001, 0.001, 0.001, 0.001, 0.0, 0.001, 0.001, 0.001, 0.0, 0.0, 0.003, 0.001, 0.001, 0.001, 0.001, 0.001, 0.001, 0.0, 0.002, 0.001, 0.001, 0.0, 0.001, 0.001, 0.002, 0.002, 0.002, 0.001]
x_centers:
[0.093, 0.1, 0.116, 0.112, 0.125, 0.101, 0.152]
y_centers:
[0.001, 0.001, 0.002, 0.001, 0.001, 0.002, 0.001]
特征 6
feature labels:
[6, 1, 1, 1, 5, 5, 3, 4, 1, 0, 1, 5, 5, 1, 1, 1, 1, 1, 4, 1, 2, 0, 1, 3, 3, 4, 2, 2, 4, 3, 3, 2, 6, 3, 1, 2, 4, 6, 1, 4, 4, 1, 4, 5, 3, 1, 1, 1, 1, 1, 0, 1, 5, 5, 1, 1, 3, 3, 3, 1, 3, 1, 3, 3, 0, 1, 2, 2, 2, 6]
x_features:
[0.0, 0.0, 0.0, 0.0, 0.0, 0.0, 0.0, 0.0, 0.0, 0.0, 0.0, 0.002, 0.0, 0.0, 0.001, 0.0, 0.001, 0.0, 0.0, 0.0, 0.0, 0.0, 0.0, 0.0, 0.0, 0.0, 0.0, 0.0, 0.001, 0.001, 0.0, 0.0, 0.0, 0.0, 0.0, 0.0, 0.0, 0.0, 0.0, 0.0, 0.001, 0.0, 0.0, 0.0, 0.0, 0.0, 0.0, 0.0, 0.0, 0.0, 0.0, 0.0, 0.001, 0.0, 0.0, 0.0, 0.0, 0.0, 0.0, 0.0, 0.0, 0.0, 0.0, 0.0, 0.0, 0.0, 0.0, 0.0, 0.0, 0.0]
y_features:
[0.0, 0.0, 0.0, 0.0, 0.0, 0.0, 0.0, 0.0, 0.0, 0.0, 0.0, 0.001, 0.0, 0.0, 0.0, 0.0, 0.0, 0.0, 0.0, 0.0, 0.0, 0.0, 0.0, 0.0, 0.0, 0.0, 0.0, 0.0, 0.0, 0.0, 0.0, 0.0, 0.0, 0.0, 0.0, 0.0, 0.0, 0.0, 0.0, 0.0, 0.0, 0.0, 0.0, 0.0, 0.0, 0.0, 0.0, 0.0, 0.0, 0.0, 0.0, 0.0, 0.0, 0.0, 0.0, 0.0, 0.0, 0.0, 0.0, 0.0, 0.0, 0.0, 0.0, 0.0, 0.0, 0.0, 0.0, 0.0, 0.0, 0.0]
x_centers:
[0.0, 0.0, 0.0, 0.0, 0.0, 0.001, 0.0]
y_centers:
[0.0, 0.0, 0.0, 0.0, 0.0, 0.0, 0.0]
在一个图上更新所有维度
根据 OP 的要求
# plot
plt.figure(figsize=(16, 8))
for j in range(0, len(centers)*2, 2):
x_features = features[:, j]
y_features = features[:, j+1]
x_centers = centers[:, j]
y_centers = centers[:, j+1]
# add the data for each label to the plot
for i, l in enumerate(labels):
plt.plot(x_features[i], y_features[i], marker=markers[int(j/2)], color=colors[int(j/2)], alpha=0.5)
# add the centers
for k in range(len(centers)):
plt.scatter(x_centers[k], y_centers[k], marker="X", color=colors[int(j/2)])
# create the rectangles for the legend
patches = [Patch(color=v, label=k) for k, v in cmap.items()]
# create centers marker for the legend
black_x = Line2D([], [], color='k', marker='X', linestyle='None', label='centers', markersize=10)
# add the legend
plt.legend(title='Labels', handles=patches + [black_x], bbox_to_anchor=(1.04, 0.5), loc='center left', borderaxespad=0, fontsize=15)
plt.show()
正如各个地块所指出的那样,有很多重叠。
添加回答
举报