1 回答
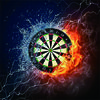
TA贡献1804条经验 获得超8个赞
您可以创建一个输入列表,在您的情况下有 2 个项目,比方说'image1'和'image2'。然后,您可以为每个以Flatten层结尾的图像创建一个卷积层和池化层的分支。
330x530 灰度图像示例:
import numpy as np
from keras.utils import plot_model
from keras.models import Model
from keras.layers import Conv2D, MaxPool2D, Flatten, Input, concatenate, Dense
inputs = [Input(shape=(330,530,1), name='image1'), Input(shape=(330,530,1), name='image2')]
flattened_layers = []
for input in inputs:
conv_layer = Conv2D(32,(3,3),activation='relu')(input)
conv_layer = MaxPool2D(pool_size=(2,2))(conv_layer)
# note that previous layer is used as input for creating the next layer,
# you'll need to do this for every layer.
# add more layers here
flattened_layers.append(Flatten()(conv_layer))
output = concatenate(flattened_layers, axis=0) #you have to check which axis you want to use here
#add more layers here
output = Dense(2,activation='softmax')(output)
model = Model(inputs=inputs, outputs=output)
model.compile(optimizer='rmsprop', loss='categorical_crossentropy', metrics=['accuracy'])
plot_model(model, "C:/help_me_pls/example_model.png", show_shapes=True)
要将数据输入此模型,您需要一个字典作为 X 值。X['image1']应包含所有第一张图片和X['image2']所有第二张图片。您应该仍然可以使用相同的 y 值。
下图显示了示例模型的架构:
我希望我已经正确理解你的问题,这就是你要找的。
添加回答
举报