3 回答
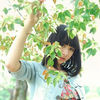
TA贡献1856条经验 获得超5个赞
我绝不是 Numpy 专家,但从我能找到的不同数组索引选项来看,这是我能找到的最快的解决方案:
bounds = np.array([[1,2], [1,3], [1,4]])
array = np.zeros((3,4))
for i, x in enumerate(bounds):
cols = slice(x[0], x[1])
array[i, cols] = 1
在这里,我们遍历边界列表并使用切片引用列。
我尝试了以下首先构建列索引列表和行索引列表的方法,但速度较慢。对于 10 000 x 10 000 阵列,在我的笔记本电脑上需要 10 秒加上 vir 0.04 秒。我猜这些切片有很大的不同。
bounds = np.array([[1,2], [1,3], [1,4]])
array = np.zeros((3,4))
cols = []
rows = []
for i, x in enumerate(bounds):
cols += list(range(x[0], x[1]))
rows += (x[1] - x[0]) * [i]
# print(cols) [1, 1, 2, 1, 2, 3]
# print(rows) [0, 1, 1, 2, 2, 2]
array[rows, cols] = 1
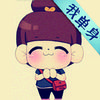
TA贡献1772条经验 获得超5个赞
解决此问题的纯 NumPy 方法的问题之一是,不存在使用轴上另一个数组的边界来“切片”NumPy 数组的方法。因此,由此产生的扩展边界最终变成了一个可变长度的列表列表,例如[[1],[1,2],[1,2,3]. 然后你可以使用np.eyeand np.sumover axis=0 来获得所需的输出。
bounds = np.array([[1,2], [1,3], [1,4]])
result = np.stack([np.sum(np.eye(4)[slice(*i)], axis=0) for i in bounds])
print(result)
array([[0., 1., 0., 0.],
[0., 1., 1., 0.],
[0., 1., 1., 1.]])
我尝试了各种方法来将np.eye(4)from [start:stop] 切片到 NumPy 的开始和停止数组,但遗憾的是,您将需要迭代来完成此操作。
编辑:另一种可以在没有任何循环的情况下以矢量化方式执行此操作的方法是-
def f(b):
o = np.sum(np.eye(4)[b[0]:b[1]], axis=0)
return o
np.apply_along_axis(f, 1, bounds)
array([[0., 1., 0., 0.],
[0., 1., 1., 0.],
[0., 1., 1., 1.]])
编辑:如果您正在寻找一个超快的解决方案但可以容忍单个 for 循环,那么根据我在该线程的所有答案中的模拟,最快的方法是-
def h(bounds):
zz = np.zeros((len(bounds), bounds.max()))
for z,b in zip(zz,bounds):
z[b[0]:b[1]]=1
return zz
h(bounds)
array([[0., 1., 0., 0.],
[0., 1., 1., 0.],
[0., 1., 1., 1.]])
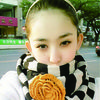
TA贡献1871条经验 获得超13个赞
使用numba.njit装饰器
import numpy as np
import numba
@numba.njit
def numba_assign_in_range(arr, bounds, val):
for i in range(len(bounds)):
s, e = bounds[i]
arr[i, s:e] = val
return arr
test_size = int(1e6) * 2
bounds = np.zeros((test_size, 2), dtype='int32')
bounds[:, 0] = 1
bounds[:, 1] = np.random.randint(0, 100, test_size)
a = np.zeros((test_size, 100))
和numba.njit
CPU times: user 3 µs, sys: 1 µs, total: 4 µs
Wall time: 6.2 µs
没有numba.njit
CPU times: user 3.54 s, sys: 1.63 ms, total: 3.54 s
Wall time: 3.55 s
添加回答
举报