1 回答
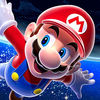
TA贡献1804条经验 获得超7个赞
DataFrame.groupby
在列上使用patient_id
并使用apply
toffill
和bfill
:
df['inclusion_timestamp'] = df.groupby('patient_id')['inclusion_timestamp']\ .apply(lambda x: x.ffill().bfill())
DataFrame.groupby
或者使用with的另一个想法Series.combine_first
:
g = df.groupby('patient_id')['inclusion_timestamp'] df['inclusion_timestamp'] = g.ffill().combine_first(g.bfill())
使用两个连续的另一个想法Series.groupby
:
df['inclusion_timestamp'] = df['inclusion_timestamp'].groupby(df['patient_id'])\ .ffill().groupby(df['patient_id']).bfill()
结果:
patient_id inclusion_timestamp pre_event_1 post_event_1 post_event_2
0 1 28-06-2020 13:05 27-06-2020 12:26 NaN NaN
1 1 28-06-2020 13:05 NaN NaN NaN
2 1 28-06-2020 13:05 NaN 29-06-2020 14:00 NaN
3 1 28-06-2020 13:05 NaN NaN 29-06-2020 23:57
4 2 29-06-2020 18:26 29-06-2020 10:11 NaN NaN
5 2 29-06-2020 18:26 NaN NaN NaN
6 2 29-06-2020 18:26 NaN 30-06-2020 19:36 NaN
7 2 29-06-2020 18:26 NaN NaN 31-06-2020 21:20
8 3 30-06-2020 09:06 29-06-2020 06:35 NaN NaN
9 3 30-06-2020 09:06 29-06-2020 07:28 NaN NaN
10 3 30-06-2020 09:06 NaN NaN NaN
11 3 30-06-2020 09:06 NaN NaN 01-07-2020 12:10
性能(使用 测量timeit):
df.shape
(1200000, 5)
%%timeit -n10 @Method 1 (Best Method)
263 ms ± 1.72 ms per loop (mean ± std. dev. of 7 runs, 10 loops each)
%%timeit -n10 @Method 2
342 ms ± 1.58 ms per loop (mean ± std. dev. of 7 runs, 10 loops each)
%%timeit -n10 @Method3
297 ms ± 4.83 ms per loop (mean ± std. dev. of 7 runs, 10 loops each)
添加回答
举报