1 回答
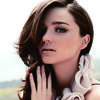
TA贡献1841条经验 获得超3个赞
我想到了!我一开始就犯了一个大错误。我需要计算
PReLU(x)=max(0,x)+a∗min(0,x)
或者 不是实际的!或者!这没有任何意义!这是普通模型(即未量化)的最终解决方案!:
torch.mintorch.max
class PReLU_2(nn.Module):
def __init__(self, prelu_object):
super().__init__()
self.prelu_weight = prelu_object.weight
self.weight = self.prelu_weight
def forward(self, inputs):
pos = torch.relu(inputs)
neg = -self.weight * torch.relu(-inputs)
inputs = pos + neg
return inputs
这是量化版本:
class PReLU_Quantized(nn.Module):
def __init__(self, prelu_object):
super().__init__()
self.prelu_weight = prelu_object.weight
self.weight = self.prelu_weight
self.quantized_op = nn.quantized.FloatFunctional()
self.quant = torch.quantization.QuantStub()
self.dequant = torch.quantization.DeQuantStub()
def forward(self, inputs):
# inputs = max(0, inputs) + alpha * min(0, inputs)
self.weight = self.quant(self.weight)
weight_min_res = self.quantized_op.mul(-self.weight, torch.relu(-inputs))
inputs = self.quantized_op.add(torch.relu(inputs), weight_min_res)
inputs = self.dequant(inputs)
self.weight = self.dequant(self.weight)
return inputs
旁注:
我在计算差异时也有错别字:
out = self.prelu(out)
out2 = self.prelu2(out)
print(f'diff : {( out - out2).mean().item()}')
out = self.conv2(out)
需要是
out1 = self.prelu(out)
out2 = self.prelu2(out)
print(f'diff : {( out1 - out2).mean().item()}')
out = self.conv2(out1)
更新:
如果您在量化方面遇到问题,您可以尝试这个版本:
import torch
import torch.nn as nn
import torch.nn.functional as F
import torch.nn.quantized as nnq
from torch.quantization import fuse_modules
class QPReLU(nn.Module):
def __init__(self, num_parameters=1, init: float = 0.25):
super(QPReLU, self).__init__()
self.num_parameters = num_parameters
self.weight = nn.Parameter(torch.Tensor(num_parameters).fill_(init))
self.relu1 = nn.ReLU()
self.relu2 = nn.ReLU()
self.f_mul_neg_one1 = nnq.FloatFunctional()
self.f_mul_neg_one2 = nnq.FloatFunctional()
self.f_mul_alpha = nnq.FloatFunctional()
self.f_add = nnq.FloatFunctional()
self.quant = torch.quantization.QuantStub()
self.dequant = torch.quantization.DeQuantStub()
self.quant2 = torch.quantization.QuantStub()
self.quant3 = torch.quantization.QuantStub()
# self.dequant2 = torch.quantization.QuantStub()
self.neg_one = torch.Tensor([-1.0])
def forward(self, x):
x = self.quant(x)
# PReLU, with modules only
x1 = self.relu1(x)
neg_one_q = self.quant2(self.neg_one)
weight_q = self.quant3(self.weight)
x2 = self.f_mul_alpha.mul(
weight_q, self.f_mul_neg_one2.mul(
self.relu2(
self.f_mul_neg_one1.mul(x, neg_one_q),
),
neg_one_q)
)
x = self.f_add.add(x1, x2)
x = self.dequant(x)
return x
m1 = nn.PReLU()
m2 = QPReLU()
# check correctness in fp
for i in range(10):
data = torch.randn(2, 2) * 1000
assert torch.allclose(m1(data), m2(data))
# toy model
class M(nn.Module):
def __init__(self):
super(M, self).__init__()
self.prelu = QPReLU()
def forward(self, x):
x = self.prelu(x)
return x
# quantize it
m = M()
m.qconfig = torch.quantization.default_qconfig
torch.quantization.prepare(m, inplace=True)
# calibrate
m(torch.randn(4, 4))
# convert
torch.quantization.convert(m, inplace=True)
# run some data through
res = m(torch.randn(4, 4))
print(res)
并确保阅读此处的注释
添加回答
举报