我正在尝试构建一个 LSTM 模型,以使用 Zipcode 或 PinCode 过去 7 天的温度、降雨量等来预测给定日期的温度。我知道训练数据集需要被塑造成(观察、时间步长、特征)。我想我的情况下的特征是温度、降雨量和时间步长为 7。因此,如果我有 2 个特征和 7 个时间步长,则一次观察总共会有 14 个变量。我还具有状态、区域(比如北、西、东、中等)等所有 7 个时间步共有的特征。由于每个观测值都是一个邮政编码,对于一个邮政编码的所有 7 天(7 个时间步长),邮政编码所属的地理州和区域将是相同的。由于我们不能向 LSTM 输入特征(对所有时间步都是通用的),我已经使用 Keras 函数式 API 将这些时间特征提供给 LSTM,并获取 LSTM 的输出并将其与非时间特征(状态、区域等)连接起来,然后在最后有一个密集层。下面是我的代码Input_LSTM = Input(shape=(7, 2), batch_size=32) # 7 timesteps and 2 featuresx = LSTM(5, stateful=True)(Input_LSTM)x = Dropout(0.2)(x)Input_MLP = Input(shape=(261,),batch_size=32) x = concatenate([x,Input_MLP], axis=1) # Join non-temporal input with LSTM o/p to be fed to a Dense # layerOutput = Dense(1)(x)model = Model([Input_LSTM,Input_MLP],Output)因为我已经声明 LSTM 是有状态的,所以我已经为时态和非时态输入提供了 batch_size。当我尝试使用行“x = concatenate([x,Input_MLP], axis=1)” 进行连接时,出现错误 File "<__array_function__ internals>", line 6, in concatenateValueError: zero-dimensional arrays cannot be concatenated”连接的两个元素的形状如下x.shapeOut[186]: TensorShape([Dimension(32), Dimension(5)]) # Here 5 is the number of neurons in LSTM, 32 is the batch sizeInput_MLP.shapeOut[187]: TensorShape([Dimension(32), Dimension(261)]) # Here 261 is the no. of non-temporal features and 32 is the batch size从网上查到零维数组是标量。但是从上面它们的形状来看,它们似乎并不是标量。我还尝试了一个以 Input_MLP 作为输入的 Dense 层,并尝试将这个 Dense 层的输出与 x 连接起来,但没有成功。我在网上找不到答案。我究竟做错了什么?任何帮助将不胜感激。
1 回答
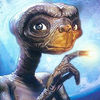
慕后森
TA贡献1802条经验 获得超5个赞
我找到了问题所在。连接 keras 层的函数必须与大写字母 C 连接。因为我使用的是带有小写字母“c”的函数,所以它是 numpy 函数,因此它给出了那个错误。一个小错字让我浪费了很多时间
添加回答
举报
0/150
提交
取消