1 回答
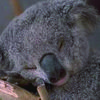
TA贡献1775条经验 获得超8个赞
我建议KarhunenLoeveSVDAlgorithm
在 OpenTURNS 中使用。它提供了 4 种随机 SVD 算法的实现。约束是必须预先设置要计算的奇异值的数量。
为了启用算法,我们必须KarhunenLoeveSVDAlgorithm-UseRandomSVD
在ResourceMap
. 然后KarhunenLoeveSVDAlgorithm-RandomSVDMaximumRank
键设置要计算的奇异值的数量(默认情况下,它等于 1000)。
提供了两种实现:
Nathan Halko、Per-Gunnar Martinsson、Joel A. Tropp。寻找具有随机性的结构:构造近似矩阵分解的概率算法,
Nathan Halko、Per-Gunnar Martisson、Yoel Shkolnisky 和 Mark Tygert。一种用于大型数据集的主成分分析的算法。
这些算法可以用KarhunenLoeveSVDAlgorithm-RandomSVDVariant
密钥来选择。
在以下示例中,我使用AbsoluteExponential
协方差模型从高斯过程中模拟了一个大型过程样本。
import openturns as ot
mesh = ot.IntervalMesher([10]*2).build(ot.Interval([-1.0]*2, [1.0]*2))
s = 0.01
model = ot.AbsoluteExponential([1.0]*2)
sampleSize = 100000
sample = ot.GaussianProcess(model, mesh).getSample(sampleSize)
然后使用随机 SVD 算法:
ot.ResourceMap_SetAsBool('KarhunenLoeveSVDAlgorithm-UseRandomSVD', True)
algorithm = ot.KarhunenLoeveSVDAlgorithm(sample, s)
algorithm.run()
result = algorithm.getResult()
该result对象包含过程的 Karhunen-Loève 分解。这对应于具有规则网格(和相等权重)的 PCA。
添加回答
举报