型。预测给出所有相同的输出验证和测试精度< 60%我尝试将最后一个隐藏层更改为softmax,但它仍然没有解决这个问题。任何反馈将不胜感激。我也尝试过使用超参数,但我仍然找不到任何修复程序。raw_cvs_data = np.loadtxt('data_to_train.csv',delimiter=',') raw_cvs_data_to_compute = np.loadtxt('data_to_compute.csv',delimiter=',') unscaled_inputs_all = raw_cvs_data[:,1:] targets_all = raw_cvs_data[:,0] inputs_to_compute = raw_cvs_data_to_compute[:] predicted_target=[] # balancing the dataset num_one_targets = int(np.sum(targets_all)) # count how many targets are 1 zero_targets_counter = 0 # counter for target 0 indices_to_remove = [] # remove extra input/target pairs for balance # count the number of targets 0, when get same amount of target 1 and 0, make entries where target is zero for i in range(targets_all.shape[0]): if targets_all[i] == 0: zero_targets_counter +=1 if zero_targets_counter > num_one_targets: indices_to_remove.append(i) unscaled_inputs_equal_priors = np.delete(unscaled_inputs_all,indices_to_remove, axis = 0) targets_equal_priors = np.delete(targets_all, indices_to_remove, axis = 0) #Shuffle the data shuffled_indices = np.arange(scaled_inputs.shape[0]) np.random.shuffle(shuffled_indices) #shuffle pairs shuffled_inputs = scaled_inputs[shuffled_indices] shuffled_targets = targets_equal_priors[shuffled_indices] # splitting data samples_count = shuffled_inputs.shape[0] # |training|validation|testing| 80-10-10 train_samples_count = int(0.8 * samples_count) validation_samples_count = int(0.1 *samples_count) test_samples_count = samples_count - train_samples_count - validation_samples_count train_inputs = shuffled_inputs[:train_samples_count] train_targets = shuffled_targets[:train_samples_count]
2 回答
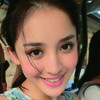
慕村225694
TA贡献1880条经验 获得超4个赞
您的问题来自以下代码行:。tf.keras.layers.Dense(output_size, activation='sigmoid')
问题是你正在使用2个神经元的“”激活,而不是1个神经元。sigmoid
使用 或 .2 neurons + activation = 'softmax'
1 neuron + activation='sigmoid'
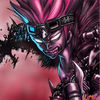
幕布斯7119047
TA贡献1794条经验 获得超8个赞
如果,看起来,您正在尝试使用单热编码标签进行分类,那么您的代码存在两个问题。
首先,你使用了错误的损失;均方误差 (MSE) 用于回归问题,而不是分类问题。更改模型编译以使用二进制交叉熵损失,即:
model.compile(optimizer='sgd', loss='binary_crossentropy', metrics=['accuracy'])
其次,正如其他答案中已经提示的那样,将最后一层的激活函数更改为 ,即:softmax
tf.keras.layers.Dense(output_size, activation='softmax')
这是单热编码标签的正确方法。
添加回答
举报
0/150
提交
取消