1 回答
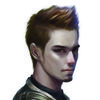
TA贡献1777条经验 获得超3个赞
None意味着它接受可变大小。
因此,您的自定义损失可以非常灵活。
实际大小自然是传递给 的数据批的大小。
如果您的数据具有形状,则无需担心。fit(samples, 128,256,3)
但是你的代码中有很多不必要的东西,你可以:
def keras_customized_loss(lambda1 = 1.0, lambda2 = 0.05):
def grad_x(image):
return K.abs(image[:, 1:] - image[:, :-1])
def grad_y(image):
return K.abs(image[:, :, 1:] - image[:, :, :-1])
def compute_loss(y_true, y_pred):
pred_grad_x = grad_x(y_pred)
pred_grad_y = grad_y(y_pred)
true_grad_x = grad_x(y_true)
true_grad_y = grad_y(y_true)
loss1 = K.mean(K.square(y_pred-y_true))
loss2 = K.mean(K.square(pred_grad_x-true_grad_x))
loss3 = K.mean(K.square(pred_grad_y-true_grad_y))
return (lambda1*loss1+lambda2*loss2+lambda2*loss3)
return compute_loss
添加回答
举报