1 回答
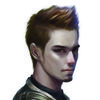
TA贡献2003条经验 获得超2个赞
据我所知,BertModel 在函数中不带标签forward()。查看forward函数参数。
我怀疑您正在尝试为序列分类任务微调 BertModel,并且 API 为BertForSequenceClassification提供了一个类。如您所见,它的 forward() 函数定义:
def forward(self, input_ids, attention_mask=None, token_type_ids=None,
position_ids=None, head_mask=None, labels=None):
请注意,forward() 方法返回以下内容。
Outputs: `Tuple` comprising various elements depending on the configuration (config) and inputs:
**loss**: (`optional`, returned when ``labels`` is provided) ``torch.FloatTensor`` of shape ``(1,)``:
Classification (or regression if config.num_labels==1) loss.
**logits**: ``torch.FloatTensor`` of shape ``(batch_size, config.num_labels)``
Classification (or regression if config.num_labels==1) scores (before SoftMax).
**hidden_states**: (`optional`, returned when ``config.output_hidden_states=True``)
list of ``torch.FloatTensor`` (one for the output of each layer + the output of the embeddings)
of shape ``(batch_size, sequence_length, hidden_size)``:
Hidden-states of the model at the output of each layer plus the initial embedding outputs.
**attentions**: (`optional`, returned when ``config.output_attentions=True``)
list of ``torch.FloatTensor`` (one for each layer) of shape ``(batch_size, num_heads, sequence_length, sequence_length)``:
Attentions weights after the attention softmax, used to compute the weighted average in the self-attention heads.
希望这可以帮助!
添加回答
举报