1 回答
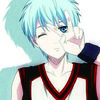
TA贡献1824条经验 获得超5个赞
根据@CodyGray 的要求,以下是工作代码。它定义了 14 * 3 = 42 个变量。OP 的代码在 for 循环中仅定义了一个 ( xA) 或三个变量 ( xA, xB, xC),这可能导致错误:duplicate key: xA.
from __future__ import print_function
import pandas as pd
from ortools.linear_solver import pywraplp
def main():
solver = pywraplp.Solver('SolveIntegerProblem',
pywraplp.Solver.CBC_MIXED_INTEGER_PROGRAMMING)
d = {
'A': [19286.0, 23786.0, 9822.0, 5054.0, 97466.0, 728998.0, 275708.0,
4576.0, 67284.0, 385582.0, 13450.0, 43271.0, 44601.0, 88372.0],
'B': [12073.0, 21563.0, 13077.0, 6407.0, 91850.0, 557996.0, 206372.0,
2812.0, 52362.0, 244102.0, 11225.0, 50612.0, 49299.0, 76099.0],
'C': [12048.0, 42648.0, 35491.0, 19800.0, 117602.0, 643498.0, 232377.0,
5217.0, 79200.0, 234259.0, 19296.0, 114048.0, 100725.0, 130911.0]
}
coeff = pd.DataFrame(data=d)
c = {
'A': [11503, 10638, 1984, 364, 15022, 40343, 41478,
238, 3528, 51649, 5759, 5305, 7883, 301],
'B': [1783, 2047, 425, 88, 2306, 6261, 6423,
51, 610, 7976, 1034, 1021, 1443, 537],
'C': [128, 250, 61, 15, 161, 453, 461,
8, 60, 566, 111, 125, 161, 57]
}
weight = pd.DataFrame(data=c)
nb_obj=len(coeff['A'])
xA = [solver.IntVar(0.0, 1.0, 'xA{:02d}'.format(i)) for i in range(nb_obj)]
xB = [solver.IntVar(0.0, 1.0, 'xB{:02d}'.format(i)) for i in range(nb_obj)]
xC = [solver.IntVar(0.0, 1.0, 'xC{:02d}'.format(i)) for i in range(nb_obj)]
# total weight per class is limited
solver.Add(sum(xA * weight.A) <= 80000)
solver.Add(sum(xB * weight.B) <= 15000)
solver.Add(sum(xC * weight.C) <= 1500)
# number of object in each class is limited
solver.Add(sum(xA) <= 3)
solver.Add(sum(xB) <= 6)
solver.Add(sum(xC) <= 5)
# 1 object can only belong to a single class
for i in range (nb_obj):
solver.Add(xA[i] + xB[i] + xC[i] == 1)
objective = solver.Objective()
for i in range(nb_obj):
objective.SetCoefficient(xA[i], coeff.A[i])
objective.SetCoefficient(xB[i], coeff.B[i])
objective.SetCoefficient(xC[i], coeff.C[i])
objective.SetMaximization()
print('Number of variables =', solver.NumVariables())
print('Number of constraints =', solver.NumConstraints())
# Solve the problem and print the solution.
result_status = solver.Solve()
# The problem has an optimal solution.
assert result_status == pywraplp.Solver.OPTIMAL
# The objective value of the solution.
print('Optimal objective value = %d' % solver.Objective().Value())
print()
# The value of each variable in the solution.
for i in range(nb_obj):
print("Obj {:02d}:".format(i), xA[i].solution_value(), xB[i].solution_value(), xC[i].solution_value())
if __name__ == '__main__':
main()
结果是:
Number of variables = 42
Number of constraints = 20
Optimal objective value = 1840645
Obj 00: 1.0 0.0 0.0
Obj 01: 0.0 1.0 0.0
Obj 02: 0.0 1.0 0.0
Obj 03: 0.0 1.0 0.0
Obj 04: 0.0 1.0 0.0
Obj 05: 0.0 0.0 1.0
Obj 06: 0.0 0.0 1.0
Obj 07: 0.0 1.0 0.0
Obj 08: 1.0 0.0 0.0
Obj 09: 1.0 0.0 0.0
Obj 10: 0.0 1.0 0.0
Obj 11: 0.0 0.0 1.0
Obj 12: 0.0 0.0 1.0
Obj 13: 0.0 0.0 1.0
添加回答
举报