2 回答
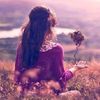
TA贡献1842条经验 获得超21个赞
这是您要划分数组的方式吗?
In [364]: arr = np.ones((1000,1000),int)
In [365]: beta = arr[25:888, 11:957]
In [366]: beta.shape
Out[366]: (863, 946)
In [367]: arr[:25,:].shape
Out[367]: (25, 1000)
In [368]: arr[888:,:].shape
Out[368]: (112, 1000)
In [369]: arr[25:888,:11].shape
Out[369]: (863, 11)
In [370]: arr[25:888,957:].shape
Out[370]: (863, 43)
我正在成像一个正方形,中间切出一个矩形。指定该矩形很容易,但必须将框架视为 4 个矩形 - 除非它是通过缺少的掩码来描述的。
检查我是否得到了一切:
In [376]: x = np.array([_366,_367,_368,_369,_370])
In [377]: np.multiply.reduce(x, axis=1).sum()
Out[377]: 1000000
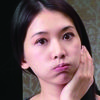
TA贡献1784条经验 获得超8个赞
假设您的原始 numpy 数组是 my_arr
提取“好”部分:
这很容易,因为好的部分是矩形的。
good_arr = my_arr[25:888, 11:957]
提取“坏”部分:
“坏”部分没有矩形形状。相反,它具有矩形的形状,从中切出一个矩形孔。
因此,您不能真正将“坏”部分单独存储在任何类似数组的结构中,除非您愿意浪费一些额外的空间来处理切出的部分。
您对“坏”部分有哪些选择?
选项 1: 对提取好的部分感到高兴和满足。让坏的部分保留为原始的一部分my_arr。在迭代 trough 时my_arr,您始终可以根据索引区分好项目和坏项目。缺点是,当你只想处理坏项时,你必须通过嵌套的双循环来完成,而不是使用 numpy 的一些矢量化功能。
选项 2: 假设我们只想对 的坏项执行一些操作,例如按行总计或按列总计my_arr,并且假设您不想要嵌套 for 循环的开销。你可以创建一个叫做 numpy 掩码数组的东西。使用屏蔽数组,您可以执行大多数常用的 numpy 操作,并且 numpy 会自动从计算中排除被屏蔽的项目。请注意,在内部,将涉及一些内存浪费,只是为了将项目存储为“屏蔽”
下面的代码说明了如何创建一个masked_arr从原始数组调用的掩码数组my_arr:
import numpy as np
my_size = 10 # In your case, 1000
r_1, r_2 = 2, 8 # In your case, r_1 = 25, r_2 = 889 (which is 888+1)
c_1, c_2 = 3, 5 # In your case, c_1 = 11, c_2 = 958 (which is 957+1)
# Using nested list comprehension, build a boolean mask as a list of lists, of shape (my_size, my_size).
# The mask will have False everywhere, except in the sub-region [r_1:r_2, c_1:c_2], which will have True.
mask_list = [[True if ((r in range(r_1, r_2)) and (c in range(c_1, c_2))) else False
for c in range(my_size)] for r in range(my_size)]
# Your original, complete 2d array. Let's just fill it with some "toy data"
my_arr = np.arange((my_size * my_size)).reshape(my_size, my_size)
print (my_arr)
masked_arr = np.ma.masked_where(mask_list, my_arr)
print ("masked_arr is:\n", masked_arr, ", and its shape is:", masked_arr.shape)
上面的输出是:
[[ 0 1 2 3 4 5 6 7 8 9]
[10 11 12 13 14 15 16 17 18 19]
[20 21 22 23 24 25 26 27 28 29]
[30 31 32 33 34 35 36 37 38 39]
[40 41 42 43 44 45 46 47 48 49]
[50 51 52 53 54 55 56 57 58 59]
[60 61 62 63 64 65 66 67 68 69]
[70 71 72 73 74 75 76 77 78 79]
[80 81 82 83 84 85 86 87 88 89]
[90 91 92 93 94 95 96 97 98 99]]
masked_arr is:
[[0 1 2 3 4 5 6 7 8 9]
[10 11 12 13 14 15 16 17 18 19]
[20 21 22 -- -- 25 26 27 28 29]
[30 31 32 -- -- 35 36 37 38 39]
[40 41 42 -- -- 45 46 47 48 49]
[50 51 52 -- -- 55 56 57 58 59]
[60 61 62 -- -- 65 66 67 68 69]
[70 71 72 -- -- 75 76 77 78 79]
[80 81 82 83 84 85 86 87 88 89]
[90 91 92 93 94 95 96 97 98 99]] , and its shape is: (10, 10)
现在你有了一个掩码数组,你将能够对其执行大部分 numpy 操作,并且 numpy 将自动排除掩码项(--打印掩码数组时显示为“ ”的项)
您可以使用掩码数组执行的操作的一些示例:
# Now, you can print column-wise totals, of only the bad items.
print (masked_arr.sum(axis=0))
# Or row-wise totals, for that matter.
print (masked_arr.sum(axis=1))
上面的输出是:
[450 460 470 192 196 500 510 520 530 540]
[45 145 198 278 358 438 518 598 845 945]
添加回答
举报