2 回答
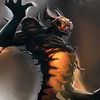
TA贡献1829条经验 获得超6个赞
毫无疑问,Hadley的干预是完美的……但是在那之后我最终对语法有所怀疑……所以,就其价值而言,我发布了完整的操作代码(对不起,我的语法与上面有些不同):
library(tidyr)
library(dplyr)
wide <-
iris %>%
mutate(row = row_number()) %>%
gather(vars, val, -Species, -row) %>%
spread(vars, val)
head(wide)
# Species row Petal.Length Petal.Width Sepal.Length Sepal.Width
# 1 setosa 1 1.4 0.2 5.1 3.5
# 2 setosa 2 1.4 0.2 4.9 3.0
# 3 setosa 3 1.3 0.2 4.7 3.2
# 4 setosa 4 1.5 0.2 4.6 3.1
# 5 setosa 5 1.4 0.2 5.0 3.6
# 6 setosa 6 1.7 0.4 5.4 3.9
head(iris)
# Sepal.Length Sepal.Width Petal.Length Petal.Width Species
# 1 5.1 3.5 1.4 0.2 setosa
# 2 4.9 3.0 1.4 0.2 setosa
# 3 4.7 3.2 1.3 0.2 setosa
# 4 4.6 3.1 1.5 0.2 setosa
# 5 5.0 3.6 1.4 0.2 setosa
# 6 5.4 3.9 1.7 0.4 setosa
他们是一样的...。只要您觉得喜欢就需要重新排序...
wide <- wide[,c(3, 4, 5, 6, 1)] ## Reorder and then remove "row" column
并做了。
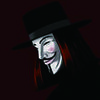
TA贡献1836条经验 获得超13个赞
由于先前的答案可能还不够清楚,因此gather您在尝试执行时会表现出自己的问题spread。
问题在于,在收集过程中,您将丢失对哪个数据feature.measure属于原始数据帧中的哪一行的了解,因此spread不知道如何再次将各个值组合到“宽”表中。
iris.df = as.data.frame(iris)
long.iris.df = iris.df %>%
tibble::rowid_to_column() %>%
gather(key = feature.measure, value = size, -Species, -rowid)
#> rowid Species feature.measure size
#> 1 1 setosa Sepal.Length 5.1
#> 2 2 setosa Sepal.Length 4.9
#> 3 3 setosa Sepal.Length 4.7
#> 4 4 setosa Sepal.Length 4.6
#> 5 5 setosa Sepal.Length 5.0
#> 6 6 setosa Sepal.Length 5.4
现在,其中的每个值都会size保留其值,rowid因此您始终可以将其重新组合到宽数据集(删除不必要的rowid):
w.iris.df = long.iris.df %>%
spread(key = feature.measure, value = size) %>%
select(-rowid)
head(w.iris.df)
#> Species Petal.Length Petal.Width Sepal.Length Sepal.Width
#> 1 setosa 1.4 0.2 5.1 3.5
#> 2 setosa 1.4 0.2 4.9 3.0
#> 3 setosa 1.3 0.2 4.7 3.2
#> 4 setosa 1.5 0.2 4.6 3.1
#> 5 setosa 1.4 0.2 5.0 3.6
#> 6 setosa 1.7 0.4 5.4 3.9
- 2 回答
- 0 关注
- 476 浏览
添加回答
举报