3 回答
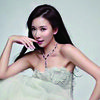
TA贡献1827条经验 获得超4个赞
IMO OP实际上并不需要np.bitwise_and()(aka &),但实际上是需要的,np.logical_and()因为它们正在比较逻辑值,例如True和False-请参阅此SO 逻辑与按位比较,以了解区别。
>>> x = array([5, 2, 3, 1, 4, 5])
>>> y = array(['f','o','o','b','a','r'])
>>> output = y[np.logical_and(x > 1, x < 5)] # desired output is ['o','o','a']
>>> output
array(['o', 'o', 'a'],
dtype='|S1')
同样的方法是np.all()通过axis适当设置参数。
>>> output = y[np.all([x > 1, x < 5], axis=0)] # desired output is ['o','o','a']
>>> output
array(['o', 'o', 'a'],
dtype='|S1')
通过数字:
>>> %timeit (a < b) & (b < c)
The slowest run took 32.97 times longer than the fastest. This could mean that an intermediate result is being cached.
100000 loops, best of 3: 1.15 µs per loop
>>> %timeit np.logical_and(a < b, b < c)
The slowest run took 32.59 times longer than the fastest. This could mean that an intermediate result is being cached.
1000000 loops, best of 3: 1.17 µs per loop
>>> %timeit np.all([a < b, b < c], 0)
The slowest run took 67.47 times longer than the fastest. This could mean that an intermediate result is being cached.
100000 loops, best of 3: 5.06 µs per loop
所以使用np.all()比较慢,但&和logical_and大致相同。
添加回答
举报