感谢您的关注 + 点赞 + 再看,对博主的肯定,会督促博主持续的输出更多的优质实战内容!!!
1.序篇-本文结构
-
背景篇-为啥需要 redis 维表
-
目标篇-做 redis 维表的预期效果是什么
-
难点剖析篇-此框架建设的难点、目前有哪些实现
-
维表实现篇-维表实现的过程
-
总结与展望篇
本文主要介绍了 flink sql redis 维表的实现过程。
如果想在本地测试下:
大数据羊说
用数据提升美好事物发生的概率~
29篇原创内容
公众号
-
在公众号后台回复flink sql 知其所以然(二)| sql 自定义 redis 数据维表获取源码(源码基于 1.13.1 实现)
-
在你的本地安装并打开 redis-server,然后使用 redis-cli 执行命令
set a "{\"score\":3,\"name\":\"namehhh\",\"name1\":\"namehhh112\"}"
-
执行源码包中的
flink.examples.sql._03.source_sink.RedisLookupTest
测试类,就可以在 console 中看到结果。
如果想直接在集群环境使用:
-
命令行执行
mvn package -DskipTests=true
打包 -
将生成的包
flink-examples-0.0.1-SNAPSHOT.jar
引入 flink lib 中即可,无需其它设置。
2.背景篇-为啥需要 redis 维表
2.1.啥是维表?事实表?
Dimension Table 概念多出现于数据仓库里面,维表与事实表相互对应。
给两个场景来看看:
比如需要统计分性别的 DAU:
-
客户端上报的日志中(事实表)只有设备 id,只用这个事实表是没法统计出分性别的 DAU 的。
-
这时候就需要一张带有设备 id、性别映射的表(这就是维表)来提供性别数据。
-
然后使用事实表去 join 这张维表去获取到每一个设备 id 对应的性别,然后就可以统计出分性别的 DAU。相当于一个扩充维度的操作。
blog.csdn.net/weixin_47482194/article/details/105855116?spm=1001.2014.3001.5501
比如目前想要统计整体销售额:
-
目前已有 “销售统计表”,是一个事实表,其中没有具体销售品项的金额。
-
“商品价格表” 可以用于提供具体销售品项的金额,这就是销售统计的一个维度表。
事实数据和维度数据的识别必须依据具体的主题问题而定。“事实表” 用来存储事实的度量及指向各个维的外键值。维表用来保存该维的元数据。
参考:blog.csdn.net/lindan1984/article/details/96566626
2.2.为啥需要 redis 维表?
目前在实时计算的场景中,熟悉 datastream 的同学大多数都使用过 mysql\Hbase\redis 作为维表引擎存储一些维度数据,然后在 datastream api 中调用 mysql\Hbase\redis 客户端去获取到维度数据进行维度扩充。
而 redis 作为 flink 实时场景中最常用的高速维表引擎,官方是没有提供 flink sql api 的 redis 维表 connector 的。如下图,基于 1.13 版本。
1
阿里云 flink 是提供了这个能力的。但是这个需要使用阿里云的产品才能使用。有钱人可以直接上。
www.alibabacloud.com/help/zh/faq-detail/122722.htm?spm=a2c63.q38357.a3.7.a1227a53TBMuSY
2
因此本文在介绍怎样自定义一个 sql 数据维表的同时,实现一个 sql redis 来给大家使用。
3.目标篇-做 redis 维表预期效果是什么
redis 作为维表在 datastream 中的最常用的数据结构就是 kv、hmap 两种。本文实现主要实现 kv 结构,map 结构大家可以拿到源码之后进行自定义实现。也就多加几行代码就完事了。
预期效果就如阿里云的 flink redis:
下面是我在本地跑的结果,先看看 redis 中存储的数据,只有这一条数据,是 json 字符串:
9
下面是预期 flink sql:
CREATE TABLE dimTable (
name STRING,
name1 STRING,
score BIGINT -- redis 中存储数据的 schema
) WITH (
'connector' = 'redis', -- 指定 connector 是 redis 类型的
'hostname' = '127.0.0.1', -- redis server ip
'port' = '6379', -- redis server 端口
'format' = 'json' -- 指定 format 解析格式
'lookup.cache.max-rows' = '500', -- guava local cache 最大条目
'lookup.cache.ttl' = '3600', -- guava local cache ttl
'lookup.max-retries' = '1' -- redis 命令执行失败后重复次数
)
SELECT o.f0, o.f1, c.name, c.name1, c.score
FROM leftTable AS o
– 维表 join
LEFT JOIN dimTable FOR SYSTEM_TIME AS OF o.proctime AS c
ON o.f0 = c.name
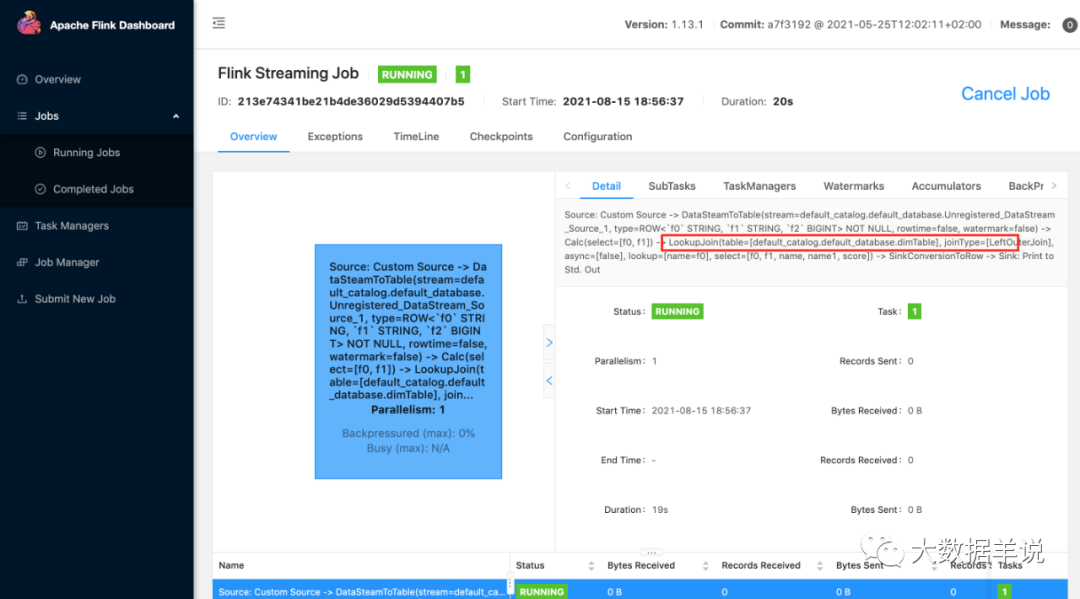
10
结果如下,后面三列就对应到 `c.name, c.name1, c.score`:
+I[a, b, namehhh, namehhh112, 3]
+I[a, b, namehhh, namehhh112, 3]
+I[a, b, namehhh, namehhh112, 3]
+I[a, b, namehhh, namehhh112, 3]
+I[a, b, namehhh, namehhh112, 3]
+I[a, b, namehhh, namehhh112, 3]
+I[a, b, namehhh, namehhh112, 3]
+I[a, b, namehhh, namehhh112, 3]
+I[a, b, namehhh, namehhh112, 3]
4.难点剖析篇-目前有哪些实现
===============
目前可以从网上搜到的实现、以及可以参考的实现有以下两个:
1. https://github.com/jeff-zou/flink-connector-redis。但是其没有实现 flink sql redis 维表,只实现了 sink 表,并且使用起来有比较多的限制,包括需要在建表时就指定 key-column,value-column 等,其实博主觉得没必要指定这些字段,这些都可以动态调整。其实现是对 apache-bahir-flink https://github.com/apache/bahir-flink 的二次开发,但与 bahir 原生实现有割裂感,因为这个项目几乎重新实现了一遍,接口也和 bahir 不同。
2. 阿里云实现 https://www.alibabacloud.com/help/zh/faq-detail/122722.htm?spm=a2c63.q38357.a3.7.a1227a53TBMuSY。可以参考的只有用法和配置等。但是有些配置项也属于阿里自定义的。
因此博主在实现时,就定了一个基调。
1. 复用 connector:复用 bahir 提供的 redis connnector
2. 复用 format:复用 flink 目前的 format 机制,目前这个上述两个实现都没有做到
3. 简洁性:实现 kv 结构。hget 封装一部分
4. 维表 local cache:为避免高频率访问 redis,维表加了 local cache 作为缓存
5.维表实现篇-维表实现的过程
===============
在实现 redis 维表之前,不得不谈谈 flink 维表加载和使用机制。
5.1.flink 维表原理
--------------
其实上节已经详细描述了 flink sql 对于 source\\sink 的加载机制,维表属于 source 的中的 lookup 表,在具体 flink 程序运行的过程之中可以简单的理解为一个 map,在 map 中调用 redis-client 接口访问 redis 进行扩充维度的过程。
1. 通过 SPI 机制加载所有的 source\\sink\\format 工厂 `Factory`
2. 过滤出 DynamicTableSourceFactory + connector 标识的 source 工厂类
3. 通过 source 工厂类创建出对应的 source
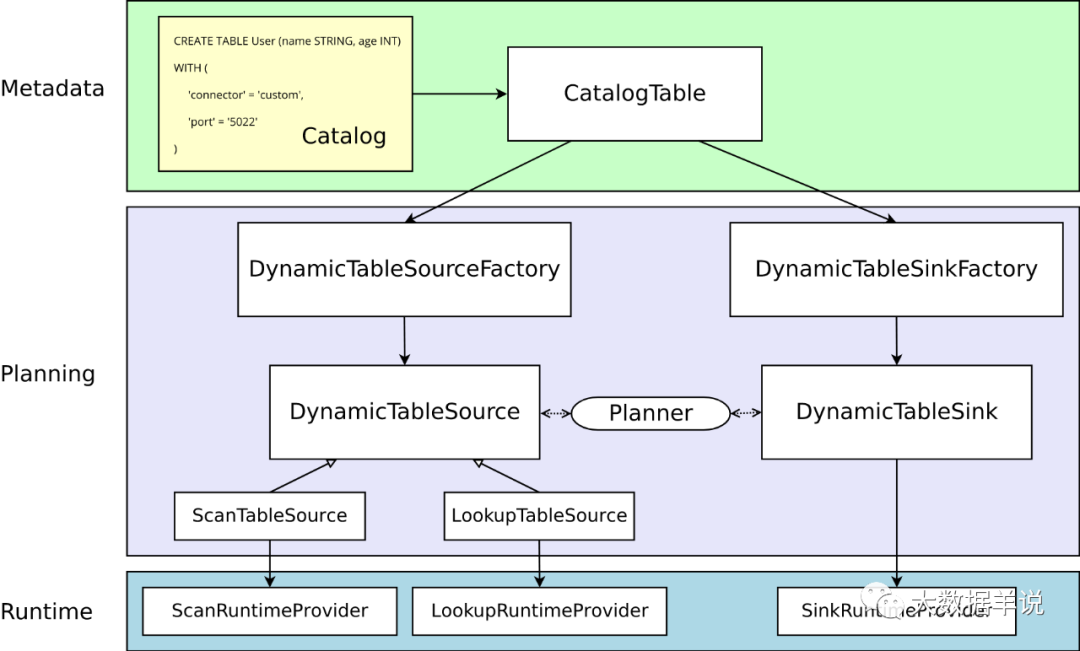
7
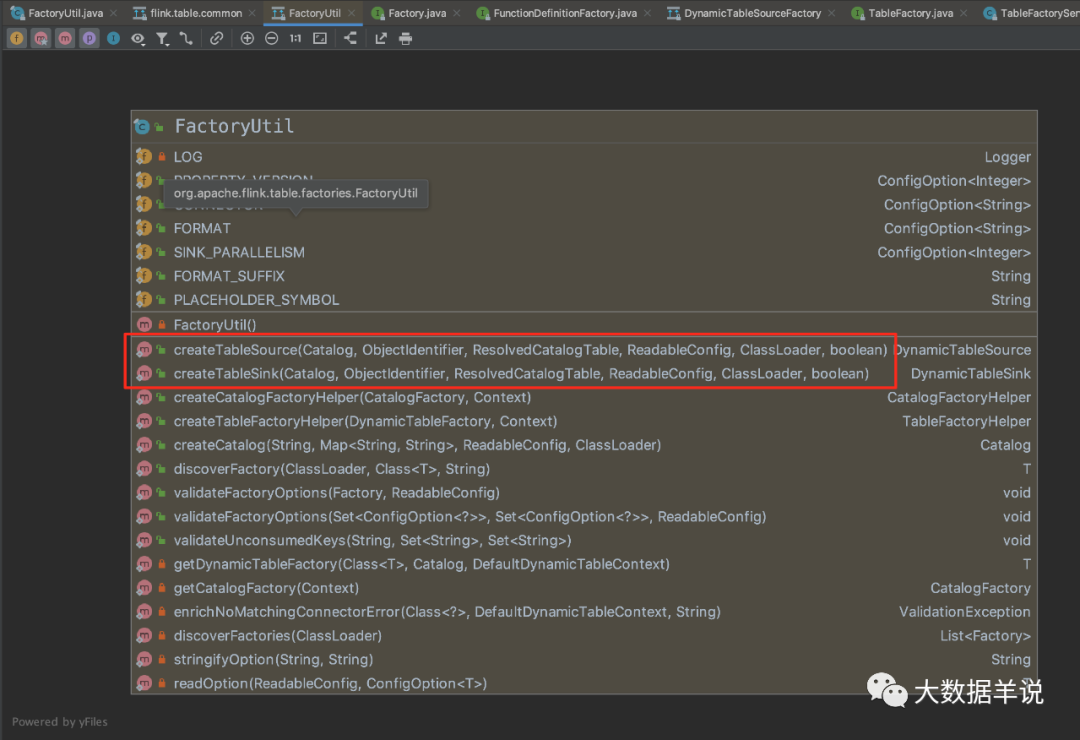
5
如图 source 和 sink 是通过 `FactoryUtil.createTableSource` 和 `FactoryUtil.createTableSink` 创建的
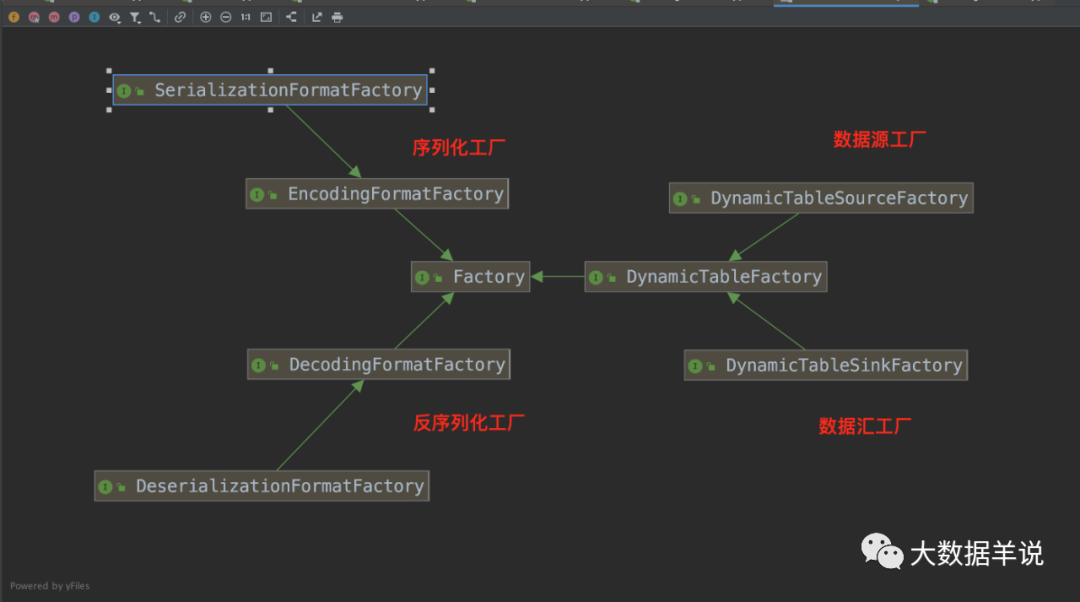
4
所有通过 SPI 的 source\\sink\\formt 插件都继承自 `Factory`。
整体创建 source 方法的调用链如下图。
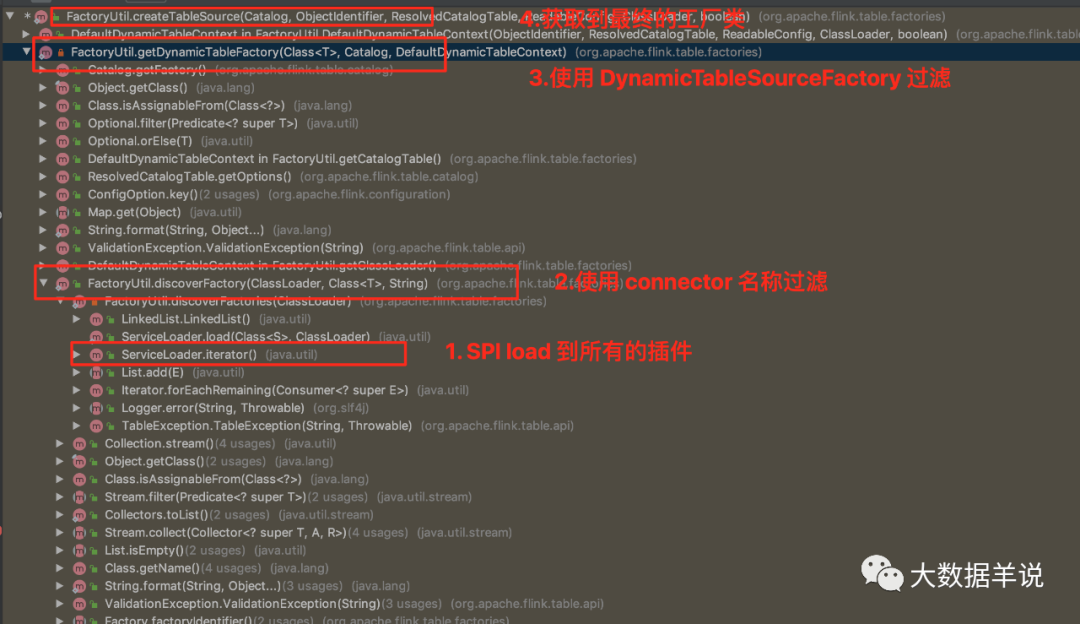
6
5.2.flink 维表实现方案
----------------
先看下博主的最终实现。
总重要的三个实现类:
1. `RedisDynamicTableFactory`
2. `RedisDynamicTableSource`
3. `RedisRowDataLookupFunction`
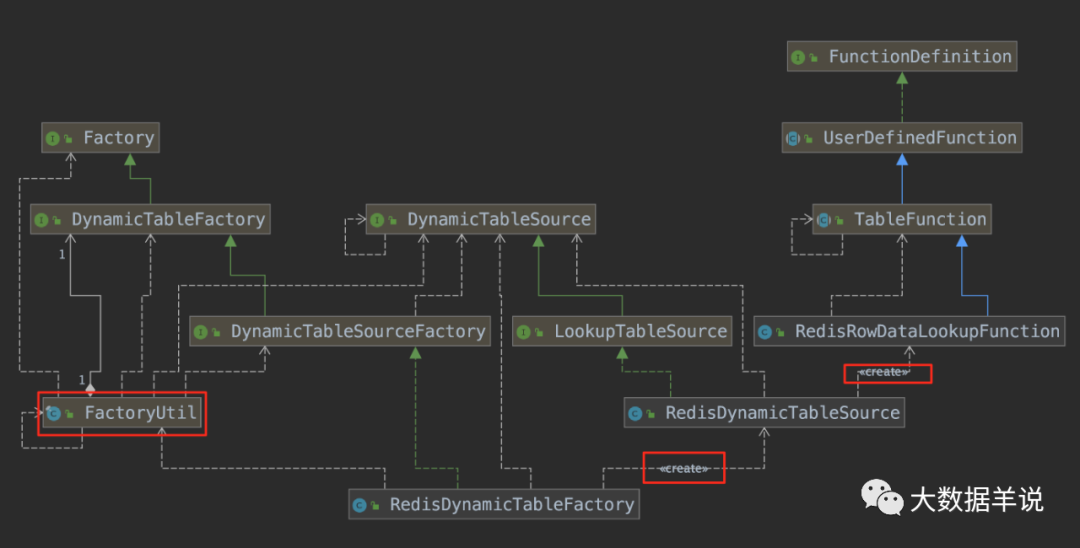
8
具体流程:
1. 定义 SPI 的工厂类 `RedisDynamicTableFactory implements DynamicTableSourceFactory`,并且在 resource\\META-INF 下创建 SPI 的插件文件
2. 实现 factoryIdentifier 标识 `redis`
3. 实现 `RedisDynamicTableFactory#createDynamicTableSource` 来创建对应的 source `RedisDynamicTableSource`
4. 定义 `RedisDynamicTableSource implements LookupTableSource`
5. 实现 `RedisDynamicTableFactory#getLookupRuntimeProvider` 方法,创建具体的维表 UDF `TableFunction<T>`,定义为 `RedisRowDataLookupFunction`
6. 实现 `RedisRowDataLookupFunction` 的 eval 方法,这个方法就是用于访问 redis 扩充维度的。
介绍完流程,进入具体实现方案细节:
`RedisDynamicTableFactory` 主要创建 source 的逻辑:
public class RedisDynamicTableFactory implements DynamicTableSourceFactory {
…
@Override
public String factoryIdentifier() {
// 标识 redis
return "redis";
}
@Override
public DynamicTableSource createDynamicTableSource(Context context) {
// either implement your custom validation logic here ...
// or use the provided helper utility
final FactoryUtil.TableFactoryHelper helper = FactoryUtil.createTableFactoryHelper(this, context);
// discover a suitable decoding format
// format 实现
final DecodingFormat<DeserializationSchema<RowData>> decodingFormat = helper.discoverDecodingFormat(
DeserializationFormatFactory.class,
FactoryUtil.FORMAT);
// validate all options
// 所有 option 配置的校验,比如 cache 类参数
helper.validate();
// get the validated options
final ReadableConfig options = helper.getOptions();
final RedisLookupOptions redisLookupOptions = RedisOptions.getRedisLookupOptions(options);
TableSchema schema = context.getCatalogTable().getSchema();
// 创建 RedisDynamicTableSource
return new RedisDynamicTableSource(
schema.toPhysicalRowDataType()
, decodingFormat
, redisLookupOptions);
}
}
resources\\META-INF 文件:
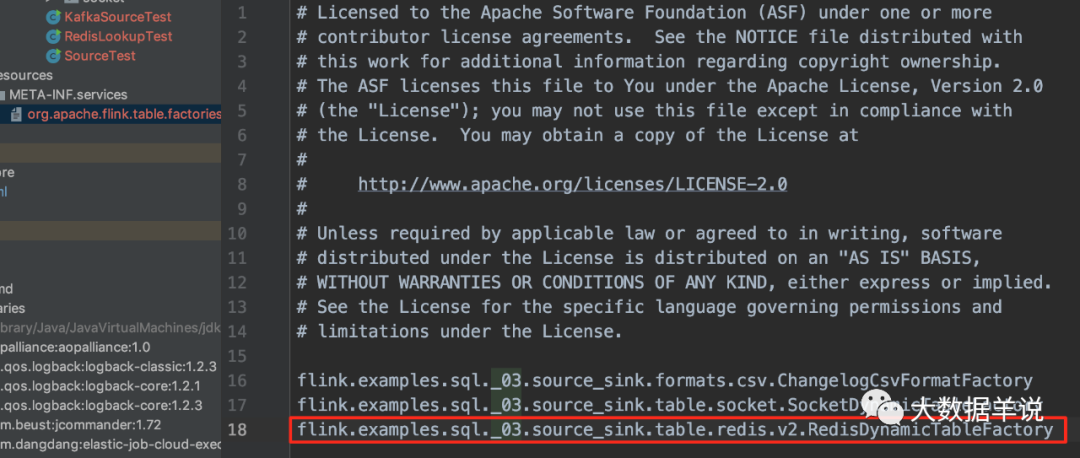
13
`RedisDynamicTableSource` 主要创建 table udf 的逻辑:
public class RedisDynamicTableSource implements LookupTableSource {
…
@Override
public LookupRuntimeProvider getLookupRuntimeProvider(LookupContext context) {
// 初始化 redis 客户端配置
FlinkJedisConfigBase flinkJedisConfigBase = new FlinkJedisPoolConfig.Builder()
.setHost(this.redisLookupOptions.getHostname())
.setPort(this.redisLookupOptions.getPort())
.build();
// redis key,value 序列化器
LookupRedisMapper lookupRedisMapper = new LookupRedisMapper(
this.createDeserialization(context, this.decodingFormat, createValueFormatProjection(this.physicalDataType)));
// 创建 table udf
return TableFunctionProvider.of(new RedisRowDataLookupFunction(
flinkJedisConfigBase
, lookupRedisMapper
, this.redisLookupOptions));
}
}
`RedisRowDataLookupFunction` table udf 执行维表关联的主要流程:
public class RedisRowDataLookupFunction extends TableFunction {
…
/**
* 具体 redis 执行方法
*/
public void eval(Object... objects) throws IOException {
for (int retry = 0; retry <= maxRetryTimes; retry++) {
try {
// fetch result
this.evaler.accept(objects);
break;
} catch (Exception e) {
LOG.error(String.format("HBase lookup error, retry times = %d", retry), e);
if (retry >= maxRetryTimes) {
throw new RuntimeException("Execution of Redis lookup failed.", e);
}
try {
Thread.sleep(1000 * retry);
} catch (InterruptedException e1) {
throw new RuntimeException(e1);
}
}
}
}
@Override
public void open(FunctionContext context) {
LOG.info("start open ...");
// redis 命令执行器,初始化 redis 链接
try {
this.redisCommandsContainer =
RedisCommandsContainerBuilder
.build(this.flinkJedisConfigBase);
this.redisCommandsContainer.open();
} catch (Exception e) {
LOG.error("Redis has not been properly initialized: ", e);
throw new RuntimeException(e);
}
// 初始化 local cache
this.cache = cacheMaxSize <= 0 || cacheExpireMs <= 0 ? null : CacheBuilder.newBuilder()
.recordStats()
.expireAfterWrite(cacheExpireMs, TimeUnit.MILLISECONDS)
.maximumSize(cacheMaxSize)
.build();
if (cache != null) {
context.getMetricGroup()
.gauge("lookupCacheHitRate", (Gauge<Double>) () -> cache.stats().hitRate());
this.evaler = in -> {
RowData cacheRowData = cache.getIfPresent(in);
if (cacheRowData != null) {
collect(cacheRowData);
} else {
// fetch result
byte[] key = lookupRedisMapper.serialize(in);
byte[] value = null;
switch (redisCommand) {
case GET:
value = this.redisCommandsContainer.get(key);
break;
case HGET:
value = this.redisCommandsContainer.hget(key, this.additionalKey.getBytes());
break;
default:
throw new IllegalArgumentException("Cannot process such data type: " + redisCommand);
}
RowData rowData = this.lookupRedisMapper.deserialize(value);
collect(rowData);
cache.put(key, rowData);
}
};
}
...
}
}
### 5.2.1.复用 bahir connector
如图是 bahir redis connector 的实现。
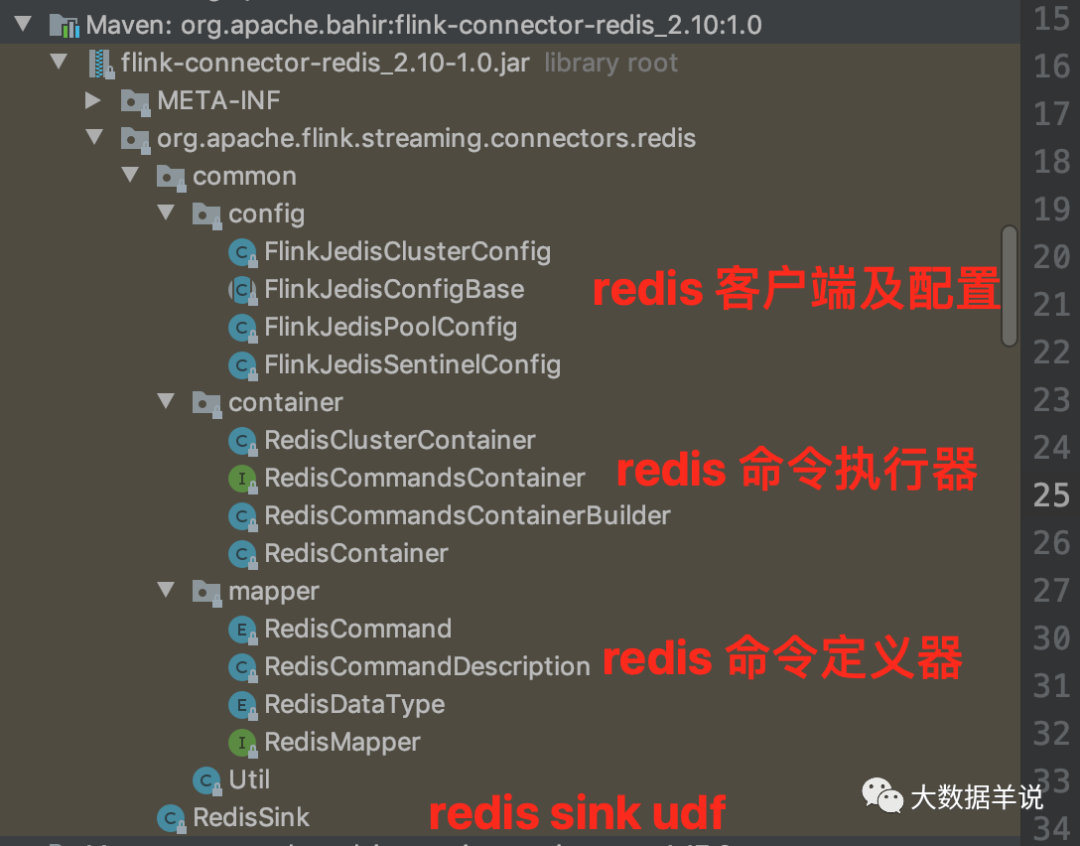
11
博主在实现过程中将能复用的都尽力复用。如图是最终实现目录。
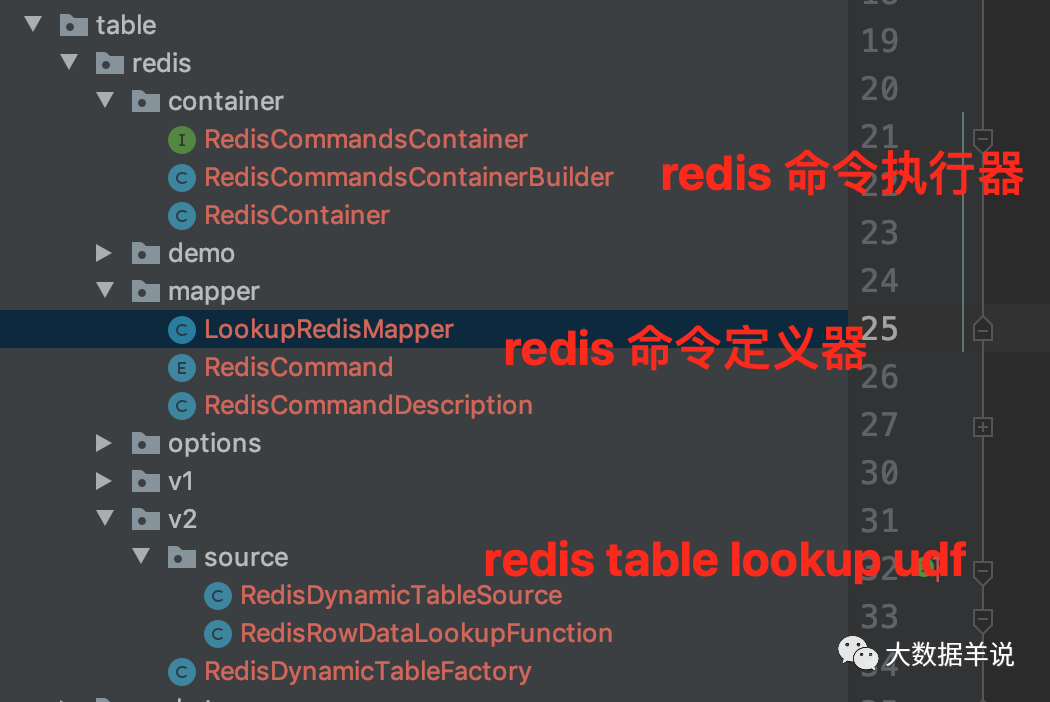
12
可以看到目录结构是与 bahir redis connector 一致的。
其中 `redis 客户端及其配置` 是直接复用了 bahir redis 的。由于 bahir redis 基本都是 sink 实现,某些实现没法继承复用,所以这里我单独开辟了目录,`redis 命令执行器` 和 `redis 命令定义器`,但是也基本和 bahir 一致。如果你想要在生产环境中进行使用,可以直接将两部分代码合并,成本很低。
### 5.2.2.复用 format
博主直接复用了 flink 本身自带的 format 机制来作为维表反序列化机制。参考 HBase connector 实现将 cache 命中率添加到 metric 中。
public class RedisDynamicTableFactory implements DynamicTableSourceFactory {
…
@Override
public DynamicTableSource createDynamicTableSource(Context context) {
…
// discover a suitable decoding format
// 复用 format 实现
final DecodingFormat<DeserializationSchema<RowData>> decodingFormat = helper.discoverDecodingFormat(
DeserializationFormatFactory.class,
FactoryUtil.FORMAT);
...
}
}
format 同样也是 SPI 机制加载。
源码公众号后台回复**flink sql 知其所以然(二)| sql 自定义 redis 数据维表**获取。
5.2.3.维表 local cache
--------------------
local cache 在初始化时可以指定 cache 大小,缓存时长等。
this.evaler = in -> {
RowData cacheRowData = cache.getIfPresent(in);
if (cacheRowData != null) {
collect(cacheRowData);
} else {
// fetch result
byte[] key = lookupRedisMapper.serialize(in);
byte[] value = null;
switch (redisCommand) {
case GET:
value = this.redisCommandsContainer.get(key);
break;
case HGET:
value = this.redisCommandsContainer.hget(key, this.additionalKey.getBytes());
break;
default:
throw new IllegalArgumentException("Cannot process such data type: " + redisCommand);
}
RowData rowData = this.lookupRedisMapper.deserialize(value);
collect(rowData);
cache.put(key, rowData);
}
};
6.总结与展望篇
========
6.1.总结
------
本文主要是针对 flink sql redis 维表进行了扩展以及实现,并且复用 bahir redis connector 的配置,具有良好的扩展性。如果你正好需要这么一个 connector,直接公众号后台回复**flink sql 知其所以然(二)| sql 自定义 redis 数据维表**获取源码吧。
6.2.展望
------
当然上述只是 redis 维表一个基础的实现,用于生产环境还有很多方面可以去扩展的。
1. jedis cluster 的扩展:目前 bahir datastream 中已经实现了,可以直接参考,扩展起来非常简单
2. aync lookup 维表的扩展:目前 hbase lookup 表已经实现了,可以直接参考实现
3. 异常 AOP,alert 等
共同学习,写下你的评论
评论加载中...
作者其他优质文章